Method for discriminating multiple waste plastic kinds by adopting near infrared spectrum characteristic wavelength
A near-infrared spectroscopy and waste plastic technology, which is applied in the direction of material analysis, analysis materials, and measurement devices by optical means, can solve the problems of complex operation process, cumbersome spectral processing process, and increased calculation time, and achieves simple analysis process. The effect of saving calculation time and reducing the amount of calculation
- Summary
- Abstract
- Description
- Claims
- Application Information
AI Technical Summary
Problems solved by technology
Method used
Image
Examples
Embodiment 1
[0039] Clean the surface of the waste plastic and polish it with 360# sandpaper, then cut it into small pieces with a size of 2mm*2mm, and finally number them and measure them by diffuse reflectance at room temperature of 20-25°C.
[0040] The present invention adopts the FT S6000 Fourier transform infrared spectrometer produced by American Bio-rad Company to collect the near-infrared spectrum of the sample. .
[0041] MATLAB was used to conduct principal component analysis on the near-infrared spectra of 86 waste plastic samples as a standard sample set after K-M transformation, and the principal component scores and loading coefficients were obtained. The cumulative contribution rate of the first four principal components reached 96.62%, which can represent the original For most of the characteristic information of the spectrum, the contribution rate of the first principal component is 75.68%, so the loading coefficient of the first principal component is the main factor, an...
Embodiment 2
[0044] With reference to the method of embodiment 1, 18 characteristic wavelength data of the plastics of 86 standard sample sets extracted are used as the input of BP neural network model, and BP neural network model is trained, and the BP neural network model input layer that training draws The number of neurons is 18, the number of neurons in the hidden layer is 20, the number of neurons in the output layer is 6, and the target error is 0.01. The transfer function of the hidden layer adopts the double tangent S-type transfer function tansig, the output layer adopts the purelin function, and the learning function trainlm is selected. The training times are 100. Then use the trained BP neural network model to predict and classify the unknown sample set. The BP neural network model has a discrimination accuracy rate of 98.84% for the standard sample set, and a prediction accuracy rate of 73.33% for the unknown sample set, which can basically meet the requirements for the class...
Embodiment 3
[0046] With reference to the method of embodiment 1, the 18 characteristic wavelength data of the plastics of 86 standard sample sets that extract are used as the input of PNN neural network model, the PNN neural network model is trained, and the dispersion constant of the PNN neural network model that training obtains is 0.1, and the maximum number of neurons in the middle layer is 86. Then use the trained PNN network model to predict and classify the unknown sample set. The PNN network training model has a classification accuracy rate of 100% for the training set and 97.67% for the prediction set, meeting the requirements for the classification of waste plastics.
PUM
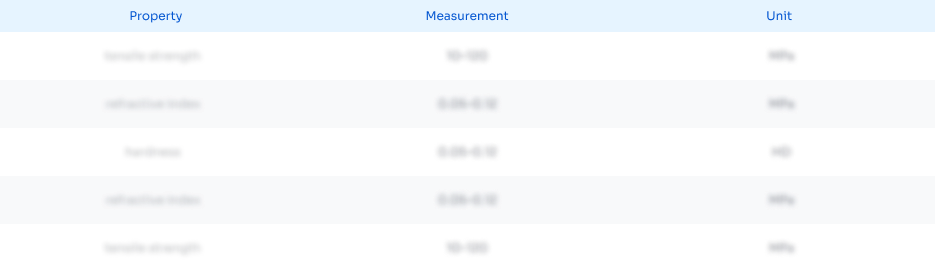
Abstract
Description
Claims
Application Information
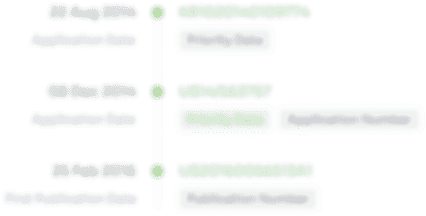
- R&D
- Intellectual Property
- Life Sciences
- Materials
- Tech Scout
- Unparalleled Data Quality
- Higher Quality Content
- 60% Fewer Hallucinations
Browse by: Latest US Patents, China's latest patents, Technical Efficacy Thesaurus, Application Domain, Technology Topic, Popular Technical Reports.
© 2025 PatSnap. All rights reserved.Legal|Privacy policy|Modern Slavery Act Transparency Statement|Sitemap|About US| Contact US: help@patsnap.com