Multi-class image semi-supervised classifying method and system
A semi-supervised, image-based technology, applied to instruments, character and pattern recognition, computer components, etc., to achieve the effects of reducing impact, improving classification accuracy, enhancing applicability and robustness
- Summary
- Abstract
- Description
- Claims
- Application Information
AI Technical Summary
Problems solved by technology
Method used
Image
Examples
Embodiment
[0046] figure 1 It is a flow chart of a multi-class image semi-supervised classification method provided by the embodiment of this application.
[0047] Such as figure 1 As shown, the method includes:
[0048] S101. Perform similarity learning on the labeled image samples and unlabeled image samples in the training set, construct a similar neighbor graph, calculate a weight coefficient matrix, and perform symmetrization and normalization processing on the weight coefficient matrix.
[0049] The embodiment of the present application provides a method for generating a training set and a test set, the method is: receiving an image sample set, and performing vectorized description on the image; selecting part of the image sample data from the image sample set after the vectorized description As a training set, the remaining image sample data is used as a test set, wherein the training set contains a small number of labeled image samples and an appropriate amount of unlabeled ima...
PUM
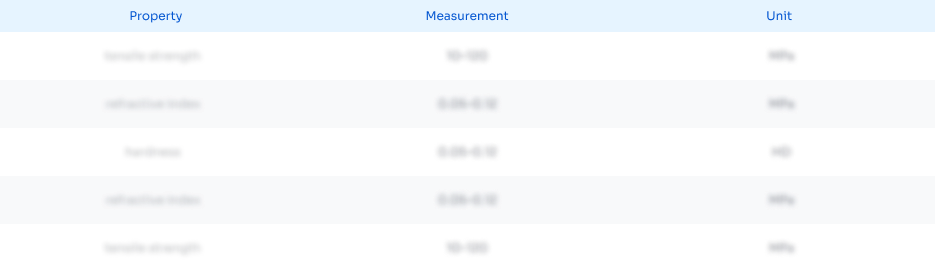
Abstract
Description
Claims
Application Information
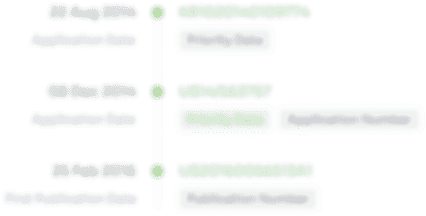
- R&D Engineer
- R&D Manager
- IP Professional
- Industry Leading Data Capabilities
- Powerful AI technology
- Patent DNA Extraction
Browse by: Latest US Patents, China's latest patents, Technical Efficacy Thesaurus, Application Domain, Technology Topic, Popular Technical Reports.
© 2024 PatSnap. All rights reserved.Legal|Privacy policy|Modern Slavery Act Transparency Statement|Sitemap|About US| Contact US: help@patsnap.com