Charged Particle Microscope Provides Depth-Resolved Images
A technology of charged particle microscopy and electrons, applied in the direction of circuits, discharge tubes, electrical components, etc., can solve problems such as not providing means to solve beam/sample interaction
- Summary
- Abstract
- Description
- Claims
- Application Information
AI Technical Summary
Problems solved by technology
Method used
Image
Examples
Embodiment 1
[0108] Figure 1A with 1B are interrelated flowcharts which show the general scheme for carrying out the method according to the invention. Referring to the nomenclature introduced in the discussion above, it is noted that:
[0109] - Figure 1A shows the iterative l For a given PSF kernel K at n algorithm. Apply sequentially for a given K n multiple iterations of the loop.
[0110] - can Figure 1A The iterative scheme in is applied sequentially to each PSF and spatial variable V. For any pair of K n , V, can perform one or more iterations in each loop. In the flow chart shown, the illustrated steps will now be explained in more detail. from Figure 1A start:
[0111] - 201: This step represents an iteration l K at n value (i.e. K n l ). exist l In the special case of =1, the previous initialization process will have been executed in order to "start" the iterative process.
[0112] -203: similarly, this step indicates an iteration l at the V value (i.e. V l ...
Embodiment 2
[0120] An intuitive way to think about the upcoming task of variable kernel deconvolution is to formulate it using so-called Bayesian statistics.
[0121] First define a number of probabilities that will be used throughout the description below:
[0122] - Pr(V|I n ) is the probability of extracting the spatial variable V, given the acquired input value I n case (see the Figure 1A Step 205 of the flow chart discussed above to obtain the value for "image" I n explanation of the concept). Similarly, Pr(I n |V) is the observed image value I given the sample structure described by V n The probability.
[0123] - Pr(V) is the so-called prior probability associated with V, representing one's knowledge about the structure to be reconstructed.
[0124] - Pr(I n ) is the probability associated with the acquired image; however, it is substantially constant, assuming the image I n is the actually observed / measured value.
[0125] Using Bayes' rule, we now get:
[0126] (1) ...
Embodiment 3
[0166] The prior distribution of the sample structure [Pr(V)] and the prior distribution of the PSF [Pr(K n )] in making the so-called posterior probability Pr(V, K n |I n ) can play an important regularization role in the process of maximization. Several well-known regularization methods are illustrated in the following publications.
[0167] [5] A. N. Tikhonov, On the Stability of Inverse Problems, Proceedings of Doklady Akademii Nauk SSSR, Russian Academy of Sciences, 1943, pp. 195-198.
[0168] [6] D. Strong, T. Chan, Edge-preserving and scale-dependent properties of total variation regularization, Inverse Problems, 2003, 19:S165–S187.
[0169] [7] P. O. Hoyer, Non-negative Matrix Factorization with Sparseness Constraints, Journal of Machine Learning Research 5, 2004, pp. 1457-1469.
[0170] [8] WD. Dong, H J. Feng, Z.H. Xu, Q. Li, A piecewise locally regularized Richardson-Lucy algorithm for remote sensing image deconvolution, Optics and Laser Technology 43, 2011, pp....
PUM
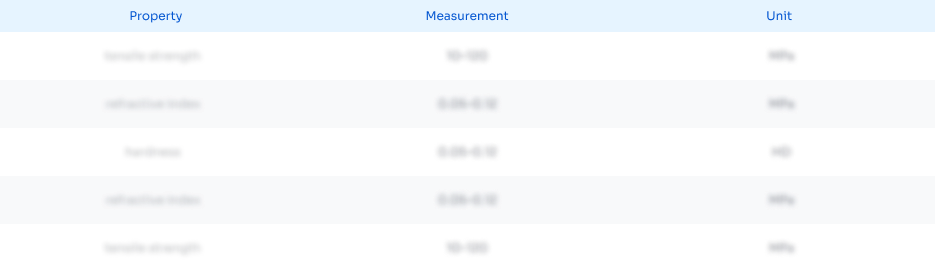
Abstract
Description
Claims
Application Information
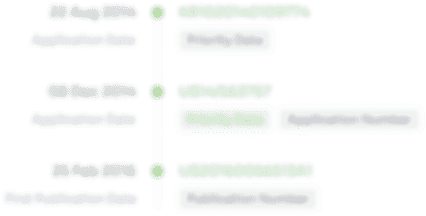
- R&D Engineer
- R&D Manager
- IP Professional
- Industry Leading Data Capabilities
- Powerful AI technology
- Patent DNA Extraction
Browse by: Latest US Patents, China's latest patents, Technical Efficacy Thesaurus, Application Domain, Technology Topic, Popular Technical Reports.
© 2024 PatSnap. All rights reserved.Legal|Privacy policy|Modern Slavery Act Transparency Statement|Sitemap|About US| Contact US: help@patsnap.com