Multi-parameter self-confirming sensor and state self-confirming method thereof
A multi-parameter, sensor technology, applied in the field of sensors
- Summary
- Abstract
- Description
- Claims
- Application Information
AI Technical Summary
Problems solved by technology
Method used
Image
Examples
specific Embodiment approach 1
[0020] Specific embodiment one: this embodiment is described in conjunction with Fig. 1, and this embodiment is made up of sensitive unit 1, traditional analysis and processing unit 2, fault diagnosis unit 3 and generation output data unit 4; Sensitive unit 1 measures a plurality of measured physical quantities, sensitive The output end of the unit 1 is respectively connected to the input end of the traditional analysis processing unit 2 and an input end of the fault diagnosis unit 3, and the other two input ends of the fault diagnosis unit 3 respectively receive the signal data of the traditional analysis processing unit 2 and other related information, The output end of the fault diagnosis unit 3 is connected to the input end of the generating output data unit 4, and the output end of the generating output data unit 4 outputs various data.
[0021] Sensing unit 1 is a measuring unit that encapsulates sensitive elements for measuring multiple parameters in one sensor and can s...
specific Embodiment approach 2
[0026] Specific implementation mode two: combination figure 2 Describe this embodiment, the steps of this embodiment are as follows:
[0027] Step 1: Input the historical data of the working state of the known sensitive unit 1 to the fault diagnosis unit 3 through other relevant information channels, use the partial least squares method to extract the principal components, and obtain various working state characteristic matrices of various sensitive units 1;
[0028] Step 2: Utilize the various working state feature matrices of the various sensitive units 1 extracted in step 1 to train the support vector classification machine, and obtain the parameters of the support vector classification machine;
[0029] Step 3: Input the measured data to the fault diagnosis unit 3 through the traditional analysis and processing unit 2, and then use the partial least squares method to extract the measured working state feature matrix, and then input the trained support vector classificatio...
specific Embodiment approach 3
[0033] Embodiment 3: The difference between this embodiment and Embodiment 1 lies in step 1. First, define the first parameter and the qth parameter data as the independent variable set X n×q , define the q+1th parameter and the mth parameter data as the data set Y n×(m-q) , n is the number of sample points, for X n×2 and Y n×1 Use the following formula (1) to standardize and get the corresponding X n×q Data set E 0 and the corresponding Y n×(m-q) Data set F 0 : (i=1, 2, ..., n; j = 1, 2, ..., m)
[0034] x ij * = x ij - x ‾ j s j - - - ( 1 )
[0035] That is, for each column of data, the mean value of the column is cu...
PUM
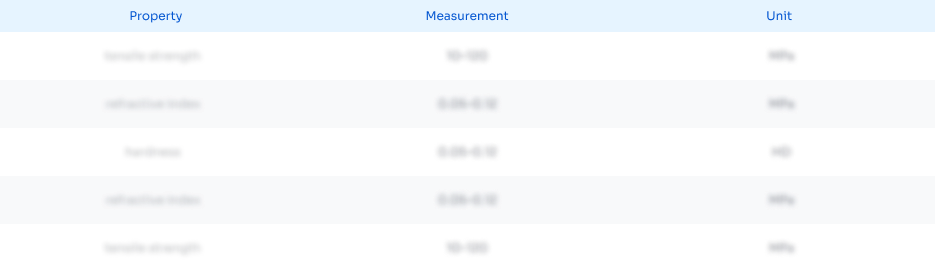
Abstract
Description
Claims
Application Information
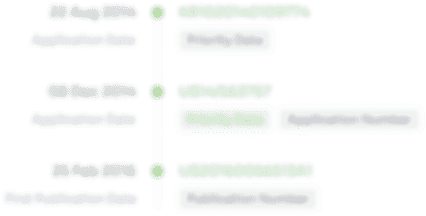
- R&D Engineer
- R&D Manager
- IP Professional
- Industry Leading Data Capabilities
- Powerful AI technology
- Patent DNA Extraction
Browse by: Latest US Patents, China's latest patents, Technical Efficacy Thesaurus, Application Domain, Technology Topic, Popular Technical Reports.
© 2024 PatSnap. All rights reserved.Legal|Privacy policy|Modern Slavery Act Transparency Statement|Sitemap|About US| Contact US: help@patsnap.com