An improved adaptive boosting algorithm based Internet intrusion detection method
An intrusion detection and Internet technology, applied in data exchange networks, digital transmission systems, electrical components, etc., can solve the problems of not meeting real-time processing requirements, difficult online retraining, and high computational complexity, and achieve easy online retraining, The effect of low false alarm rate and low computational complexity
- Summary
- Abstract
- Description
- Claims
- Application Information
AI Technical Summary
Problems solved by technology
Method used
Image
Examples
Embodiment 1
[0045] Embodiment 1: That is, it is actually applied in the intrusion detection system of the present invention. For each feature dimension, all training samples are used to construct a weak classifier according to Bayesian rule. In this way, a total of 41 weak classifiers can be obtained, that is, the size of the generated weak classifier group is 41.
[0046]Since different feature dimensions have different properties, some are continuous features and some are discrete features, different processing methods should be adopted when applying Bayesian rule, which will be described separately below.
[0047] 1) Design a weak classifier for continuous features
[0048] Let the training sample x i marked as y i ∈{+1,-1}, the value of a continuous feature f is x if . We want to find a value θ in the value domain of this feature * To optimally split the value domain, that is:
[0049] θ * = arg min ...
Embodiment 2
[0061] Embodiment 2: Select 3 features from 41 features and combine them together, randomly select a subset from the training sample set for each combination, and use the support vector machine algorithm to obtain a weak classifier. Then, you can get a total of C 41 3 = 21320 a weak classifier. That is, the size of the generated weak classifier group is 21320.
Embodiment 3
[0062] Embodiment 3: The weak classifier group may not be generated in advance, but may be generated in each cycle of the improved Adaboost algorithm. The sample weight of the current cycle of the improved Adaboost algorithm is used as an estimate of the probability of the sample, and according to the C4.5 algorithm of the decision tree, all values of each feature under all 41-dimensional features are examined to find the optimal split point to generate The next level of tree nodes. In general, we split the decision tree at no more than three levels.
[0063] According to the present invention, the step of generating a strong classifier: on the basis of the step of generating a weak classifier, use the improved Adaboost algorithm to select a part of weak classifiers from the weak classifier group and calculate the weight of the weak classifiers, and integrate the strong Classifier.
[0064] Using the improved Adaboost algorithm, that is, in each cycle, the current optima...
PUM
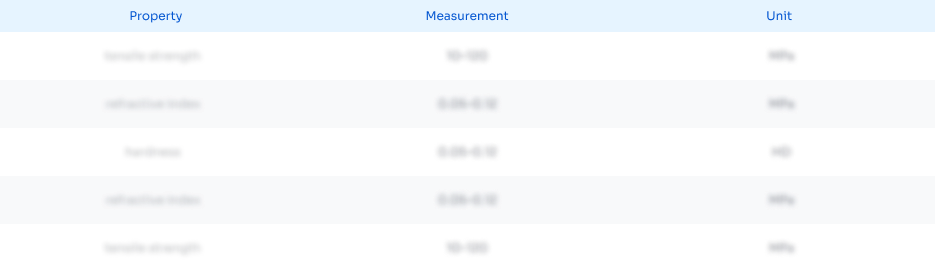
Abstract
Description
Claims
Application Information
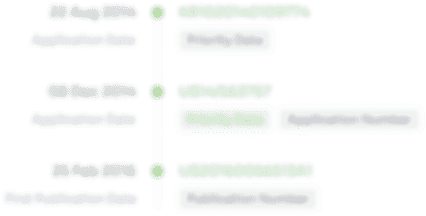
- R&D Engineer
- R&D Manager
- IP Professional
- Industry Leading Data Capabilities
- Powerful AI technology
- Patent DNA Extraction
Browse by: Latest US Patents, China's latest patents, Technical Efficacy Thesaurus, Application Domain, Technology Topic, Popular Technical Reports.
© 2024 PatSnap. All rights reserved.Legal|Privacy policy|Modern Slavery Act Transparency Statement|Sitemap|About US| Contact US: help@patsnap.com