Multi-view image classification method based on hierarchical graph enhanced stacked auto-encoder
A technology of stacking self-encoders and self-encoders, which is applied in the field of multi-view image classification based on graph enhancement, can solve problems such as the inability to extract multi-view image features, and achieve the effect of maintaining geometric structure, balancing complementarity and consistency
- Summary
- Abstract
- Description
- Claims
- Application Information
AI Technical Summary
Problems solved by technology
Method used
Image
Examples
Embodiment 1
[0054] like figure 1 As shown, this embodiment provides a multi-view image classification method based on a layered graph enhanced stacked autoencoder, including the following steps:
[0055] Step S1, sample collection
[0056] Collect multi-view samples χ={X (1) , X (2) ,...,X (V) }, and normalize it;
[0057] in N is the number of samples, d v is the dimension of the vth view, and V represents the number of views;
[0058] Step S2, build a model
[0059] Build an autoencoder network model, which includes an autoencoder and a fully connected neural network; let the parameters of the vth view in the autoencoder be The parameters in the fully connected neural network are Initialize the parameters of all views in the autoencoder and parameters in a fully connected neural network and public representation H:
[0060] where l represents the lth layer of the autoencoder, L represents the total number of layers of the autoencoder; m represents the mth layer of the f...
Embodiment 2
[0089] This embodiment also provides a multi-view image classification system based on a layered graph enhanced stacked autoencoder, including a sample collection module, a model model building, a model training module, and a real-time classification module, specifically:
[0090] The sample collection module is used to collect multi-view samples χ={X (1) , X (2) ,...,X (V) }, and normalize it;
[0091] in N is the number of samples, d v is the dimension of the vth view, and V represents the number of views;
[0092] Build a model model for building an autoencoder network model. The autoencoder network model includes an autoencoder and a fully connected neural network; let the parameters of the vth view in the autoencoder be The parameters in the fully connected neural network are Initialize the parameters of all views in the autoencoder and the parameters in the fully connected neural network and public representation H;
[0093] where l represents the lth layer...
Embodiment 3
[0120] This embodiment also provides a computer device, including a memory and a processor, where a computer program is stored in the memory, and when the computer program is executed by the processor, the processor enables the processor to execute the above-mentioned multi-view image based on the layered graph enhanced stacked autoencoder The steps of the classification method.
[0121] Wherein, the computer device may be a desktop computer, a notebook computer, a palmtop computer, a cloud server and other computing devices. The computer device can perform human-computer interaction with the user through a keyboard, a mouse, a remote control, a touch pad or a voice control device.
[0122] The memory includes at least one type of readable storage medium, and the readable storage medium includes flash memory, hard disk, multimedia card, card-type memory (for example, SD or D interface display memory, etc.), random access memory (RAM), Static random access memory (SRAM), read ...
PUM
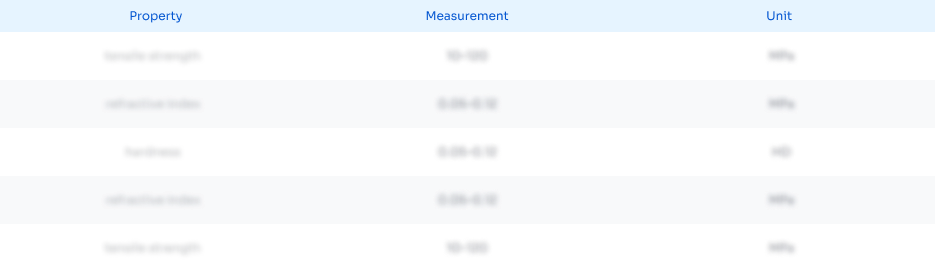
Abstract
Description
Claims
Application Information
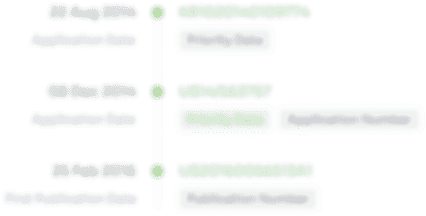
- Generate Ideas
- Intellectual Property
- Life Sciences
- Materials
- Tech Scout
- Unparalleled Data Quality
- Higher Quality Content
- 60% Fewer Hallucinations
Browse by: Latest US Patents, China's latest patents, Technical Efficacy Thesaurus, Application Domain, Technology Topic, Popular Technical Reports.
© 2025 PatSnap. All rights reserved.Legal|Privacy policy|Modern Slavery Act Transparency Statement|Sitemap|About US| Contact US: help@patsnap.com