Bearing fault diagnosis method and system based on improved deep residual algorithm
A fault diagnosis and fault diagnosis model technology, applied in mechanical bearing testing, neural learning methods, calculations, etc., can solve problems such as low precision and low efficiency, and achieve the effects of good extraction, strong generalization ability, and low time cost
- Summary
- Abstract
- Description
- Claims
- Application Information
AI Technical Summary
Problems solved by technology
Method used
Image
Examples
Embodiment 1
[0053] In the first aspect, the present invention first proposes a bearing fault diagnosis method based on an improved depth residual algorithm, see figure 1 , the method includes:
[0054] S1. Convert the acquired one-dimensional time series signal of bearing fault into two-dimensional image data, and form a fault data set based on the two-dimensional image data;
[0055] S2. A Resnet-SK bearing fault diagnosis model constructed based on the fault data set training;
[0056] The Resnet-SK bearing fault diagnosis model includes: adding an improved SK attention mechanism to the shortcut branch of the BasicBlock structure of the deep residual network, and modifying the second conventional convolution on the residual branch of the BasicBlock structure to A dilated convolution with an expansion rate of 2; the improved SK attention mechanism includes: using a multi-scale dilated convolution group to replace the two branch convolutions in the split stage of the SK attention mechani...
Embodiment 2
[0085] In a second aspect, the present invention also provides a bearing fault diagnosis system based on an improved depth residual algorithm, the system comprising:
[0086] a data set acquisition module, configured to convert the acquired one-dimensional time series signal of bearing fault into two-dimensional image data, and form a fault data set based on the two-dimensional image data;
[0087] A model training module, which is used to train the Resnet-SK bearing fault diagnosis model constructed based on the fault data set;
[0088] The Resnet-SK bearing fault diagnosis model includes: adding an improved SK attention mechanism to the shortcut branch of the BasicBlock structure of the deep residual network, and replacing the second conventional convolution on the residual branch of the BasicBlock structure with A dilated convolution with an expansion rate of 2; the improved SK attention mechanism includes: using a multi-scale dilated convolution group to replace the two br...
PUM
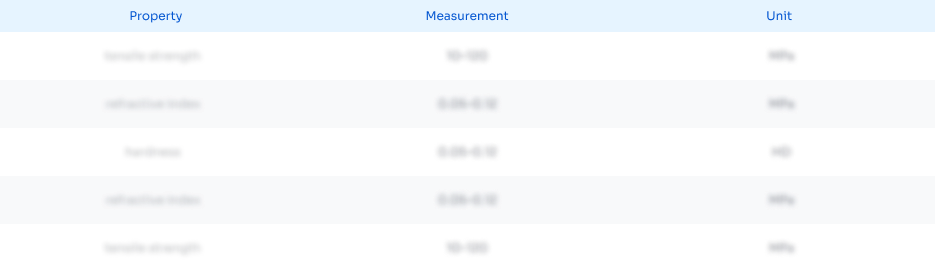
Abstract
Description
Claims
Application Information
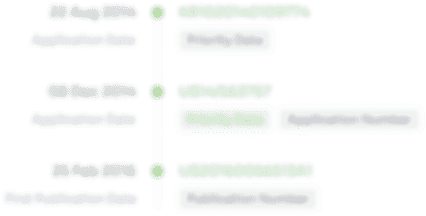
- R&D Engineer
- R&D Manager
- IP Professional
- Industry Leading Data Capabilities
- Powerful AI technology
- Patent DNA Extraction
Browse by: Latest US Patents, China's latest patents, Technical Efficacy Thesaurus, Application Domain, Technology Topic, Popular Technical Reports.
© 2024 PatSnap. All rights reserved.Legal|Privacy policy|Modern Slavery Act Transparency Statement|Sitemap|About US| Contact US: help@patsnap.com