CBCT alveolar bone segmentation system and method based on deep learning
A technology of deep learning and alveolar bone, which is applied in the field of artificial intelligence medical image processing and orthodontics, can solve the problems of blurred boundaries, differences in alveolar bone between layers, and low accuracy of threshold segmentation method, and achieve highly automated alveolar bone Segmentation, the effect of high-precision alveolar bone segmentation
- Summary
- Abstract
- Description
- Claims
- Application Information
AI Technical Summary
Problems solved by technology
Method used
Image
Examples
Embodiment example 1
[0051] like figure 1 As shown, the present invention provides a technical scheme: a deep learning-based CBCT alveolar bone segmentation system and method, comprising the following steps:
[0052] S1. Dental CBCT data set acquisition and labeling: collect multiple patient CBCT images, and then use ITK-SNAP software to label the alveolar bone;
[0053] S2, CBCT image and labeled sample preprocessing: normalize the sample and store it as a specific data structure;
[0054] S3. Construction of deep semantic segmentation model: U-shaped semantic segmentation network model based on Swin-transformer and skip connection;
[0055] S4. Model training and evaluation: Use the CrossEntropyLoss and Diceloss loss functions to evaluate the model training effect;
[0056] S5. Dental CBCT data segmentation and reconstruction.
specific Embodiment example 2
[0058] S1. Dental CBCT data set acquisition and labeling
[0059] S11. Obtain the CBCT image data of 10 patients from the dental hospital, and the CBCT data of each patient is a collection of 512 512x512 dcm format images;
[0060]S12, such as figure 2 As shown, the alveolar bone annotation was performed on all images of each patient's CBCT dataset using the ITK-SNAP software, the label files were saved in the nii file format, and the CBCT images and labels were visualized.
[0061] S2, CBCT image and labeled sample preprocessing
[0062] S21. Convert the DICOM format of the CBCT image to PNG format, and then normalize the image to correct the grayscale to be between 0 and 255. The normalization formula is as follows:
[0063] x'=(x-min(cbct)) / (max(cbct)-min(cbct))
[0064] where min(cbct) is the minimum gray value of each CBCT dataset; max(cbct) is the maximum gray value of each CBCT dataset;
[0065] S22. Extract the 2D slice images in turn from the 3D nii annotation fi...
PUM
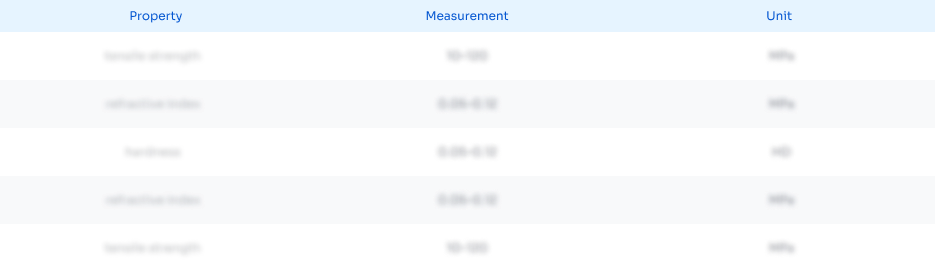
Abstract
Description
Claims
Application Information
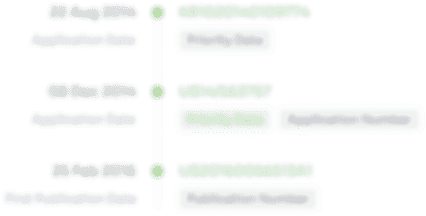
- R&D Engineer
- R&D Manager
- IP Professional
- Industry Leading Data Capabilities
- Powerful AI technology
- Patent DNA Extraction
Browse by: Latest US Patents, China's latest patents, Technical Efficacy Thesaurus, Application Domain, Technology Topic, Popular Technical Reports.
© 2024 PatSnap. All rights reserved.Legal|Privacy policy|Modern Slavery Act Transparency Statement|Sitemap|About US| Contact US: help@patsnap.com