Transferable image adversarial sample generation and deep neural network test method and system
A technology of image samples and adversarial samples, applied in biological neural network models, neural learning methods, neural architectures, etc., can solve problems such as weak ability to find errors, poor image sample quality, unstable optimization direction, etc., and achieve the success rate of generation High, increase diversity, ensure the effect of generating quality
- Summary
- Abstract
- Description
- Claims
- Application Information
AI Technical Summary
Problems solved by technology
Method used
Image
Examples
Embodiment Construction
[0046] Below in conjunction with specific embodiments, the present invention will be further illustrated, and it should be understood that these embodiments are only used to illustrate the present invention and not to limit the scope of the present invention. The modifications all fall within the scope defined by the appended claims of this application.
[0047] like figure 1 As shown, a method for generating a transferable image adversarial sample disclosed in an embodiment of the present invention mainly includes: first, setting relevant parameter information, including the maximum disturbance change value ε, the maximum number of iterations T, and the types of disturbance radii of neighboring image samples. The number N, the number of adjacent image samples M on each radius, the radius coefficient β, the maximum scale coefficient inter, the attenuation coefficient μ, and the generated disturbance step size λ=ε / T, the magnification coefficient of the adjacent image sample di...
PUM
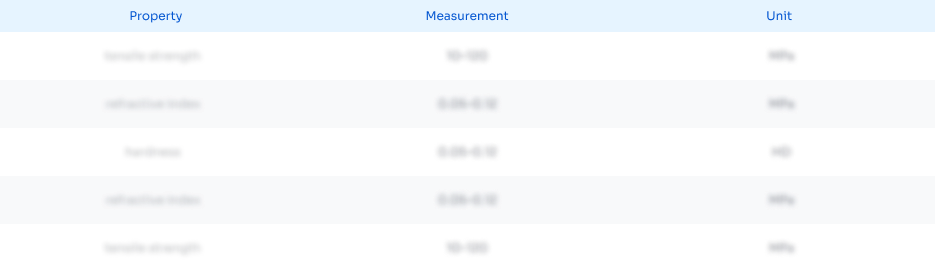
Abstract
Description
Claims
Application Information
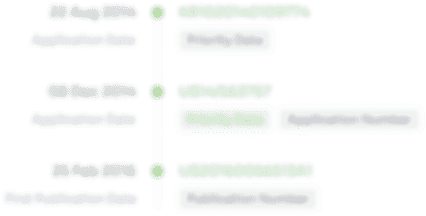
- R&D Engineer
- R&D Manager
- IP Professional
- Industry Leading Data Capabilities
- Powerful AI technology
- Patent DNA Extraction
Browse by: Latest US Patents, China's latest patents, Technical Efficacy Thesaurus, Application Domain, Technology Topic, Popular Technical Reports.
© 2024 PatSnap. All rights reserved.Legal|Privacy policy|Modern Slavery Act Transparency Statement|Sitemap|About US| Contact US: help@patsnap.com