Fuel cell voltage prediction method based on long short-term memory neural network model
A neural network model, long-term and short-term memory technology, applied in the direction of biological neural network model, neural learning method, neural architecture, etc., can solve the problems of difficulty and difficulty in classification of working conditions, achieve early perception, improve accuracy, and improve adaptability sexual effect
- Summary
- Abstract
- Description
- Claims
- Application Information
AI Technical Summary
Problems solved by technology
Method used
Image
Examples
Embodiment Construction
[0057] The present invention will be further described below with reference to the accompanying drawings and embodiments.
[0058] like figure 1 As shown, a fuel cell voltage prediction method based on a long short-term memory neural network model includes offline training and online detection stages;
[0059] Offline training phase:
[0060] Step 1: Obtain various historical detection signals of the fuel cell, including voltage, current, inlet water temperature, outlet water temperature, cooling water pressure, hydrogen pressure, air outlet pressure, air inlet pressure, air flow, and air temperature.
[0061] Step 2: Preprocess the historical detection signal obtained in Step 1 to decompose the predicted voltage signal.
[0062] In step 2, the predicted voltage signal of the fuel cell is obtained according to the following formula:
[0063] V_pre(n)=V_measure(n+1)
[0064] Among them: V_pre is the predicted voltage signal, V_measure is the measured voltage signal, n is th...
PUM
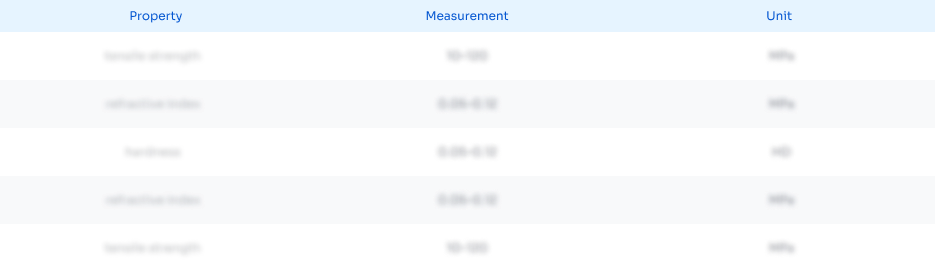
Abstract
Description
Claims
Application Information
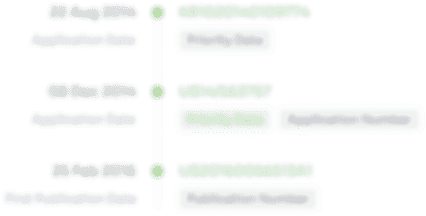
- R&D Engineer
- R&D Manager
- IP Professional
- Industry Leading Data Capabilities
- Powerful AI technology
- Patent DNA Extraction
Browse by: Latest US Patents, China's latest patents, Technical Efficacy Thesaurus, Application Domain, Technology Topic, Popular Technical Reports.
© 2024 PatSnap. All rights reserved.Legal|Privacy policy|Modern Slavery Act Transparency Statement|Sitemap|About US| Contact US: help@patsnap.com