A Contrastive Self-Supervised Learning Method Based on Multi-Network Framework
A supervised learning and multi-network technology, applied in neural learning methods, biological neural network models, instruments, etc., can solve problems such as poor sample recognition ability, increased network parameters, increased training time, etc., to improve network model performance and enhance training effects, performance-enhancing effects
- Summary
- Abstract
- Description
- Claims
- Application Information
AI Technical Summary
Problems solved by technology
Method used
Image
Examples
Embodiment Construction
[0026] In order to make the content of the present invention easier to understand clearly, the present invention will be described in further detail below with reference to the accompanying drawings.
[0027] like figure 1 As shown, a comparative self-supervised learning method based on a multi-network framework, the steps are:
[0028] Step 1. Perform data augmentation on each image in the training set to obtain three independent augmented views;
[0029] Perform data augmentation on the images in the training set. The data augmentation strategies used include random cropping and resizing, horizontal flipping, color distortion and grayscale conversion, and random Gaussian blurring is applied to the above data;
[0030] Step 2. Input the three augmented views into the backpropagation network, the stop gradient network and the momentum network respectively to obtain the corresponding image representation;
[0031] Among them, the backpropagation network includes an encoder and ...
PUM
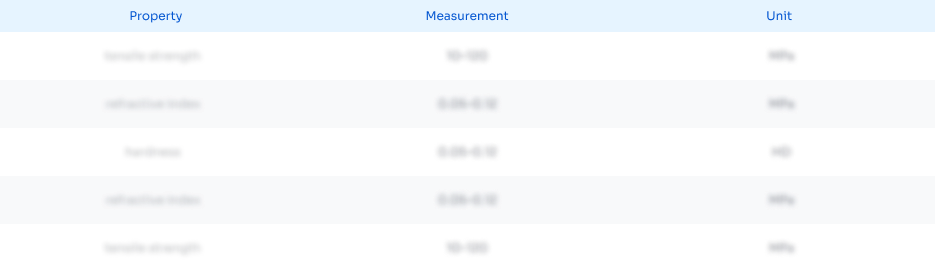
Abstract
Description
Claims
Application Information
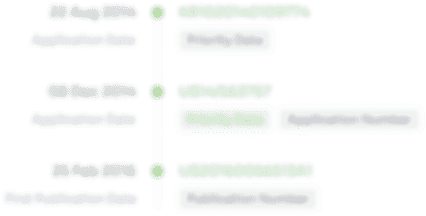
- Generate Ideas
- Intellectual Property
- Life Sciences
- Materials
- Tech Scout
- Unparalleled Data Quality
- Higher Quality Content
- 60% Fewer Hallucinations
Browse by: Latest US Patents, China's latest patents, Technical Efficacy Thesaurus, Application Domain, Technology Topic, Popular Technical Reports.
© 2025 PatSnap. All rights reserved.Legal|Privacy policy|Modern Slavery Act Transparency Statement|Sitemap|About US| Contact US: help@patsnap.com