Abnormal driving behavior online identification method based on Encoder-Decoder attention network and LSTM
A technology of abnormal driving and identification method, applied in the field of online identification of abnormal driving behavior, can solve the problems of high installation and maintenance costs, consume large computing resources, and limited application scenarios, and achieve low cost, promote accurate identification, and avoid interference Effect
- Summary
- Abstract
- Description
- Claims
- Application Information
AI Technical Summary
Problems solved by technology
Method used
Image
Examples
Embodiment Construction
[0052] An online identification method of abnormal driving behavior based on Encoder-Decoder attention network and LSTM. The specific steps are:
[0053] Step 1. Obtain mobile phone sensor data, and perform preprocessing and normal distribution transformation to obtain driving behavior time series data;
[0054]The present invention considers various abnormal driving behaviors, and it is difficult for a single sensor to capture various driving behaviors. Therefore, the abnormal driving behavior is described by means of multi-sensor fusion, wherein the accelerometer data reflects the sudden acceleration / deceleration situation to a certain extent, Gyroscope data can be used to analyze sharp turns, and the movement caused by rapid lane changes can be recorded by multiple sensors. At the same time, there is a significant coupling between the abnormal driving behaviors. In view of this, the present invention combines different sensor data at the same time to form a driving behavio...
PUM
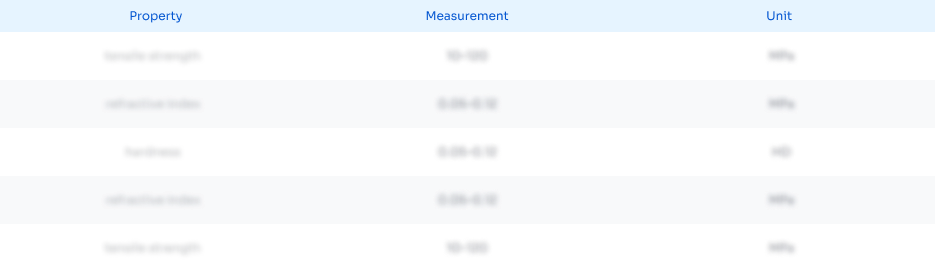
Abstract
Description
Claims
Application Information
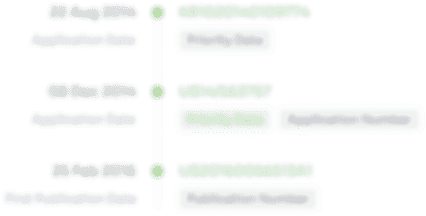
- Generate Ideas
- Intellectual Property
- Life Sciences
- Materials
- Tech Scout
- Unparalleled Data Quality
- Higher Quality Content
- 60% Fewer Hallucinations
Browse by: Latest US Patents, China's latest patents, Technical Efficacy Thesaurus, Application Domain, Technology Topic, Popular Technical Reports.
© 2025 PatSnap. All rights reserved.Legal|Privacy policy|Modern Slavery Act Transparency Statement|Sitemap|About US| Contact US: help@patsnap.com