Vehicle flow prediction method combining attention mechanism and dynamic space-time convolution model
A technology of vehicle flow and convolution model, which is applied in traffic flow detection, road vehicle traffic control system, traffic control system, etc. It can solve the problems such as the inability to effectively utilize the transfer relationship of road traffic flow and the inability to capture dynamic changes in spatial dependencies. achieve the effect of improving accuracy
- Summary
- Abstract
- Description
- Claims
- Application Information
AI Technical Summary
Problems solved by technology
Method used
Image
Examples
Embodiment Construction
[0021] The following will clearly and completely describe the technical solutions in the embodiments of the present invention with reference to the accompanying drawings in the embodiments of the present invention. Obviously, the described embodiments are only some, not all, embodiments of the present invention. Based on the embodiments of the present invention, all other embodiments obtained by persons of ordinary skill in the art without making creative efforts belong to the protection scope of the present invention.
[0022] The road electric bicycle traffic prediction method that combines the attention mechanism and the dynamic space-time graph convolution model proposed by the present invention will be described in detail below. The execution process of the method is as follows figure 1 shown.
[0023] For the convenience of description, the definition of related symbols and the definition of road flow prediction problem are given:
[0024] Road network graph is defined ...
PUM
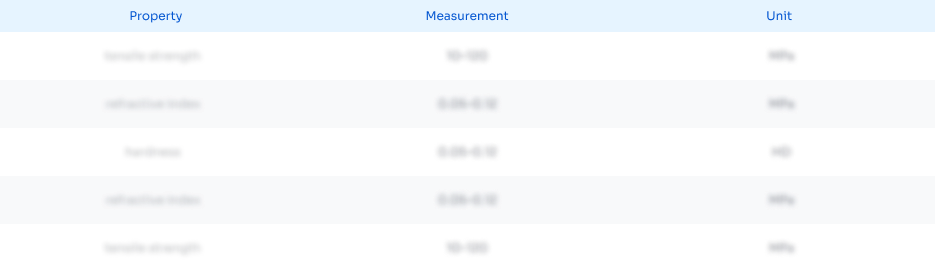
Abstract
Description
Claims
Application Information
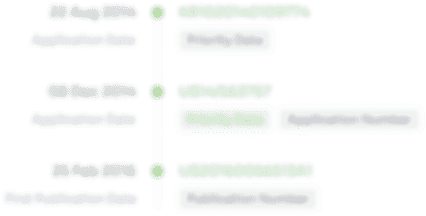
- R&D Engineer
- R&D Manager
- IP Professional
- Industry Leading Data Capabilities
- Powerful AI technology
- Patent DNA Extraction
Browse by: Latest US Patents, China's latest patents, Technical Efficacy Thesaurus, Application Domain, Technology Topic, Popular Technical Reports.
© 2024 PatSnap. All rights reserved.Legal|Privacy policy|Modern Slavery Act Transparency Statement|Sitemap|About US| Contact US: help@patsnap.com