A method for predicting the remaining life of marine bearings based on transfer learning and multiple time windows
A technology of life prediction and transfer learning, applied in neural learning methods, character and pattern recognition, instruments, etc., can solve the problems of inaccurate prediction of bearing remaining life and difficulty in adapting to multiple degradation modes, so as to improve the efficiency of fault identification and improve High prediction accuracy and high prediction efficiency
- Summary
- Abstract
- Description
- Claims
- Application Information
AI Technical Summary
Problems solved by technology
Method used
Image
Examples
Embodiment Construction
[0065] In order to make the object, technical solution and advantages of the present invention clearer, the present invention will be further described in detail below in conjunction with the accompanying drawings and embodiments. It should be understood that the specific embodiments described here are only used to explain the present invention, not to limit the present invention. In addition, the technical features involved in the various embodiments of the present invention described below can be combined with each other as long as they do not constitute a conflict with each other.
[0066] The technical problems to be solved by the present invention are: 1. Due to individual differences in bearings and differences in the types of failures that occur, it is difficult for the life prediction model trained by the traditional method to obtain a single degradation mode to achieve a good remaining life prediction effect under other degradation modes; 2. For the rolling bearing da...
PUM
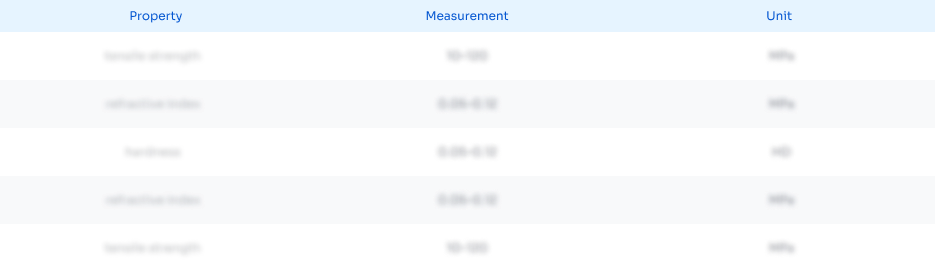
Abstract
Description
Claims
Application Information
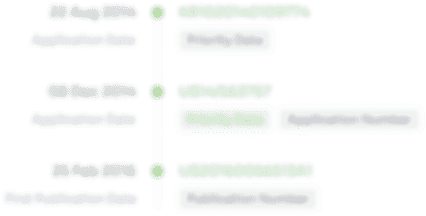
- R&D Engineer
- R&D Manager
- IP Professional
- Industry Leading Data Capabilities
- Powerful AI technology
- Patent DNA Extraction
Browse by: Latest US Patents, China's latest patents, Technical Efficacy Thesaurus, Application Domain, Technology Topic, Popular Technical Reports.
© 2024 PatSnap. All rights reserved.Legal|Privacy policy|Modern Slavery Act Transparency Statement|Sitemap|About US| Contact US: help@patsnap.com