Honeycomb lung focus segmentation method based on SAA-Unet network
A honeycomb lung and network technology, applied in the field of image processing, can solve the problems of complex texture, large deformation, irregular shape, etc., and achieve the effect of accurate segmentation
- Summary
- Abstract
- Description
- Claims
- Application Information
AI Technical Summary
Problems solved by technology
Method used
Image
Examples
Embodiment Construction
[0029] Such as Figure 1 to Figure 3 As shown, the cellular lung lesion segmentation method based on the SAA-Unet network provided by the present invention is specifically carried out according to the following steps:
[0030] Step S1: Obtain CT image data of patients with cellulite lung in different age groups, perform binarization, feature labeling, etc., and perform image enhancement and other preprocessing operations on the images to realize the expansion of the data set;
[0031] Step S2: Divide the training set, test set and verification set by preset ratios to fully verify the generalization ability of the model;
[0032] Step S3: Construct the basic U-Net network, and replace the Softmax activation function with 1×1 convolution and Sigmoid activation function in the last layer of the network;
[0033] Step S4: Improve the constructed basic U-Net network to obtain an improved U-Net network based on divided attention and attention mechanism, and use the divided attentio...
PUM
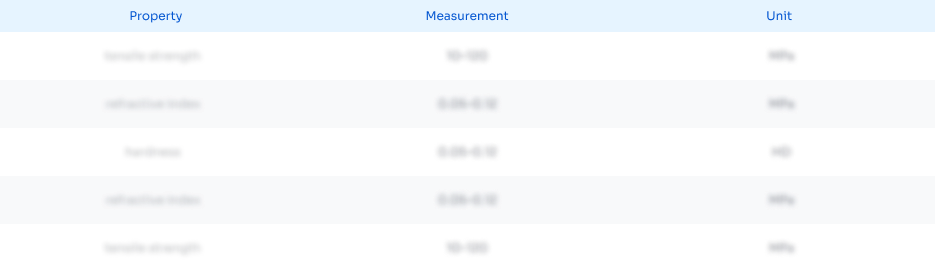
Abstract
Description
Claims
Application Information
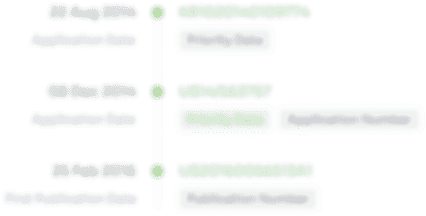
- Generate Ideas
- Intellectual Property
- Life Sciences
- Materials
- Tech Scout
- Unparalleled Data Quality
- Higher Quality Content
- 60% Fewer Hallucinations
Browse by: Latest US Patents, China's latest patents, Technical Efficacy Thesaurus, Application Domain, Technology Topic, Popular Technical Reports.
© 2025 PatSnap. All rights reserved.Legal|Privacy policy|Modern Slavery Act Transparency Statement|Sitemap|About US| Contact US: help@patsnap.com