Graph convolutional neural network pooling method based on adaptive filtering
A convolutional neural network and adaptive filtering technology, applied in neural learning methods, biological neural network models, neural architectures, etc., can solve problems such as inability to identify pharmacophore, and achieve high classification accuracy, better comprehensive performance, and comprehensive Excellent performance
- Summary
- Abstract
- Description
- Claims
- Application Information
AI Technical Summary
Problems solved by technology
Method used
Image
Examples
Embodiment Construction
[0024] The technical solutions of the present invention are further explained below with reference to the embodiments and the accompanying drawings, but this is not intended to limit the protection scope of the present application.
[0025] The present invention provides a graph convolutional neural network pooling method (method for short) based on adaptive filtering, which includes the following two parts:
[0026] The first part, the convolution operation based on Self-adaptive Filtering with Graph Convolutional Networks (SFGCN): design low-pass filters, high-pass filters and band-pass filters in the frequency domain, and combine each The filter is converted into the corresponding spatial domain convolution kernel; each spatial domain convolution kernel is convolved through a graph convolutional neural network to obtain the embedding matrix corresponding to each filter; each embedding matrix is summed to obtain the total embedding matrix;
[0027] 1) Design the low-pass f...
PUM
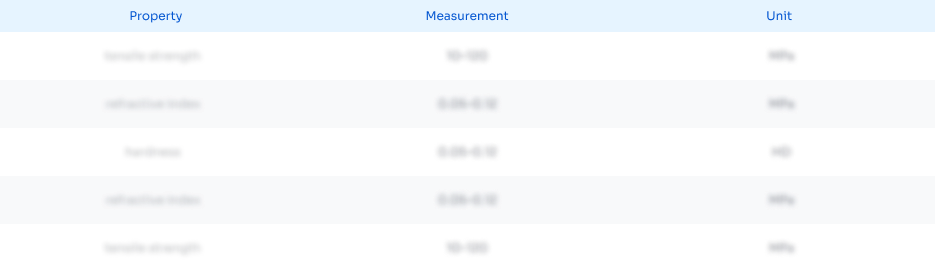
Abstract
Description
Claims
Application Information
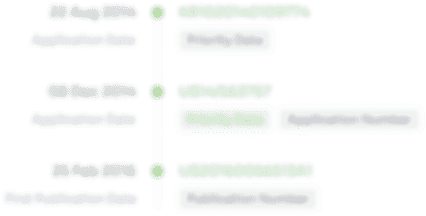
- R&D Engineer
- R&D Manager
- IP Professional
- Industry Leading Data Capabilities
- Powerful AI technology
- Patent DNA Extraction
Browse by: Latest US Patents, China's latest patents, Technical Efficacy Thesaurus, Application Domain, Technology Topic, Popular Technical Reports.
© 2024 PatSnap. All rights reserved.Legal|Privacy policy|Modern Slavery Act Transparency Statement|Sitemap|About US| Contact US: help@patsnap.com