Multi-level feature fused generative adversarial network image defogging method
A network image, generative technology, applied in the field of image processing, can solve the problems of dull color, easy to fail calculation in the sky area of the image, easy distortion of the image edge, etc. The effect of robustness
- Summary
- Abstract
- Description
- Claims
- Application Information
AI Technical Summary
Problems solved by technology
Method used
Image
Examples
Embodiment Construction
[0034] The technical solution of the present invention will be specifically described below in conjunction with the accompanying drawings.
[0035] The present invention is a generative confrontation network image defogging method that integrates multi-level features, embeds the structure of U-Net into the generator of the generative confrontation network, and proposes a generative confrontation network that integrates multi-level features, including for The generator that generates the defogged image, the discriminator used to discriminate the defogged image and the label image and feed back the result to the generator; Feature extraction is performed separately, and the extracted different feature maps are learned through the SE-ResNet module; then, the learned multi-level feature maps are spliced to fuse more image features; then, when upsampling, the fused The feature map is put into the SE module for learning to better allocate channel weights and enhance useful feature...
PUM
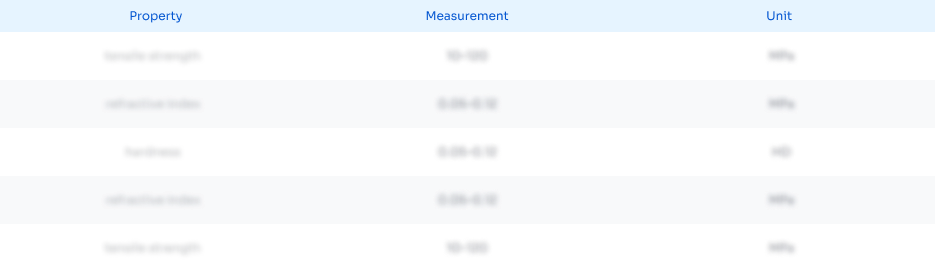
Abstract
Description
Claims
Application Information
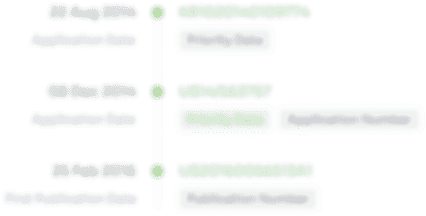
- R&D Engineer
- R&D Manager
- IP Professional
- Industry Leading Data Capabilities
- Powerful AI technology
- Patent DNA Extraction
Browse by: Latest US Patents, China's latest patents, Technical Efficacy Thesaurus, Application Domain, Technology Topic, Popular Technical Reports.
© 2024 PatSnap. All rights reserved.Legal|Privacy policy|Modern Slavery Act Transparency Statement|Sitemap|About US| Contact US: help@patsnap.com