Semi-supervised learning image segmentation method, system and terminal
A semi-supervised learning and image segmentation technology, applied in neural learning methods, image analysis, image enhancement, etc., can solve problems such as time-consuming and labor-intensive
- Summary
- Abstract
- Description
- Claims
- Application Information
AI Technical Summary
Problems solved by technology
Method used
Image
Examples
Embodiment Construction
[0026] The scheme will be described below in conjunction with the accompanying drawings and specific implementation methods.
[0027] figure 1 For a schematic flow chart of an image segmentation method for semi-supervised learning provided in the embodiment of the present application, see figure 1 , the image segmentation method of the semi-supervised learning in the present embodiment comprises:
[0028] S101. Use the first dataset of labeled images to train an image segmentation network.
[0029] In this embodiment, X represents a grayscale image, and Y represents its corresponding label image. The method uses two datasets, one is a dataset D with labeled images L ={X L ,Y L}, where the label image Y L is the ground-truth annotation from experts on the grayscale image X L The manual segmentation; the other is an unlabeled image dataset D U ={X U}, there are only grayscale images X in this dataset U , and its corresponding label is unknown. By training the 3D scSE-...
PUM
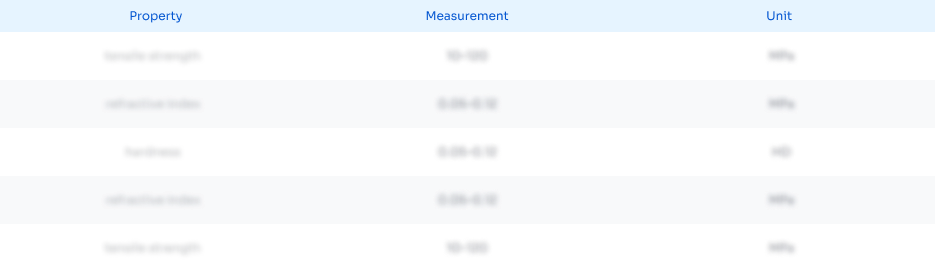
Abstract
Description
Claims
Application Information
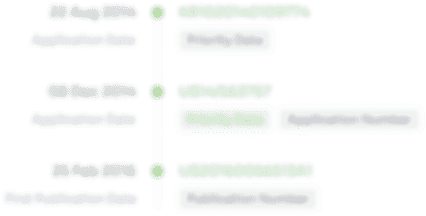
- Generate Ideas
- Intellectual Property
- Life Sciences
- Materials
- Tech Scout
- Unparalleled Data Quality
- Higher Quality Content
- 60% Fewer Hallucinations
Browse by: Latest US Patents, China's latest patents, Technical Efficacy Thesaurus, Application Domain, Technology Topic, Popular Technical Reports.
© 2025 PatSnap. All rights reserved.Legal|Privacy policy|Modern Slavery Act Transparency Statement|Sitemap|About US| Contact US: help@patsnap.com