Vibration image-driven rolling bearing intelligent fault diagnosis method
A technology for rolling bearing and fault diagnosis, which is applied in EMD-AADPC vibration image and CNN rolling bearing fault diagnosis, in the field of rolling bearing fault diagnosis, can solve the problems of single characteristic information, unclear characteristics of vibration image samples, and enhanced sample characteristics, etc., to achieve image High resolution, realization of mechanical fault diagnosis, comprehensive effect of signal characteristics
- Summary
- Abstract
- Description
- Claims
- Application Information
AI Technical Summary
Problems solved by technology
Method used
Image
Examples
Embodiment example
[0073] 1) The convolutional network model structure used in the experiment is as follows: Figure 4 As shown, the total number of layers is 7 layers, including 3 layers of convolutional layers, 2 layers of pooling layers, and 2 layers of fully connected layers. Under the condition that the image sample information is not affected by the image color, the use of a single-channel image can reduce network input parameters, reduce the proportion of memory usage, and improve network computing efficiency. Therefore, the sample size used in the present invention is 256*256. channel grayscale image, vibrating image samples such as Figure 5 As shown; the output is 4 nodes, that is, the 4 types of bearing status categories. During the training process, the hyperparameters in the model are fine-tuned through each training and verification evaluation results. The initial model parameters are shown in Table 1.
[0074] Table 1 Initial parameters of the model
[0075]
[0076]
[007...
PUM
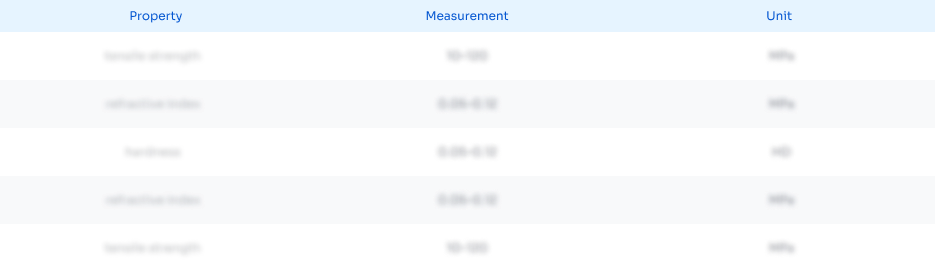
Abstract
Description
Claims
Application Information
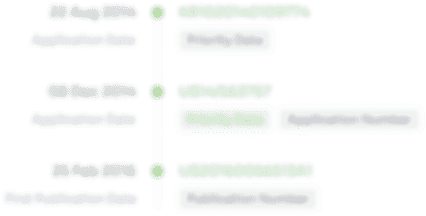
- R&D Engineer
- R&D Manager
- IP Professional
- Industry Leading Data Capabilities
- Powerful AI technology
- Patent DNA Extraction
Browse by: Latest US Patents, China's latest patents, Technical Efficacy Thesaurus, Application Domain, Technology Topic, Popular Technical Reports.
© 2024 PatSnap. All rights reserved.Legal|Privacy policy|Modern Slavery Act Transparency Statement|Sitemap|About US| Contact US: help@patsnap.com