Low-rank tensor completion method based on three-dimensional total variation and Tucker decomposition
A fully variable, three-dimensional technology, applied in the field of digital image processing, can solve problems such as losing high-dimensional data space structure, and achieve efficient solution results
- Summary
- Abstract
- Description
- Claims
- Application Information
AI Technical Summary
Problems solved by technology
Method used
Image
Examples
Embodiment Construction
[0057] The present invention will be described in further detail below in conjunction with specific embodiments and accompanying drawings.
[0058] A low-rank tensor completion method based on 3DTV and Tucker decomposition, including the following steps:
[0059] Step 1, use MATLAB software to read in the damaged video file with high loss rate, and process it into a three-dimensional tensor tensor The size is X×Y×M;
[0060] Step 2, the kernel tensor in the target functional of tensor completion and factor matrix by tensor Decomposed, so it is not conducive to the solution of the target functional, need to introduce three auxiliary variables, namely the matrix and tensors and tensor At this time, the target functional is transformed into formula (3),
[0061]
[0062] In formula (3), the adjustment parameter λ 1 and lambda 2 , balancing the weights between 3DTV and low-rank constraints, where λ 1 >0,λ 2 >0,D w is the weighted three-dimensional differenc...
PUM
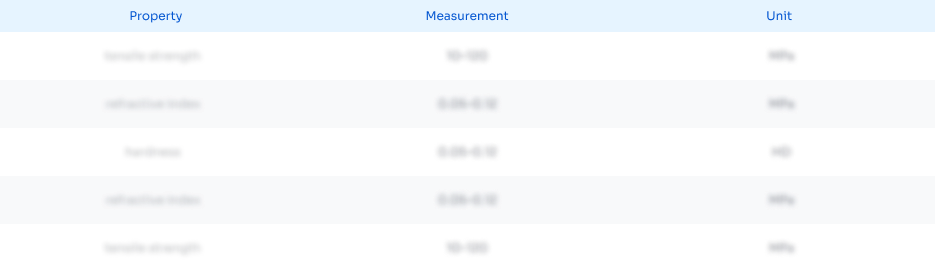
Abstract
Description
Claims
Application Information
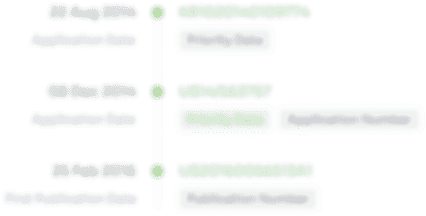
- R&D Engineer
- R&D Manager
- IP Professional
- Industry Leading Data Capabilities
- Powerful AI technology
- Patent DNA Extraction
Browse by: Latest US Patents, China's latest patents, Technical Efficacy Thesaurus, Application Domain, Technology Topic, Popular Technical Reports.
© 2024 PatSnap. All rights reserved.Legal|Privacy policy|Modern Slavery Act Transparency Statement|Sitemap|About US| Contact US: help@patsnap.com