Medical image recognition method based on Knet deep learning
A technology of medical imaging and recognition methods, applied in neural learning methods, character and pattern recognition, image analysis, etc., which can solve problems such as occupying computer operating memory and running time, insufficient accuracy of pathological judgment, and inaccurate segmentation of abnormal images, etc. , to achieve the effect of increasing the efficiency of recognition operation, improving the efficiency of judgment operation, and improving the accuracy of diagnosis
- Summary
- Abstract
- Description
- Claims
- Application Information
AI Technical Summary
Problems solved by technology
Method used
Image
Examples
Embodiment Construction
[0040] The specific embodiment of the present invention will be further described below in conjunction with accompanying drawing and specific embodiment:
[0041] Such as figure 1 and figure 2 As shown, this medical image recognition method based on K_net deep learning, where K_net includes an input layer, an encoder, a decoder, and an output prediction result that are progressively linked by logical processing order, and the encoder contains multiple layers that are progressively linked from top to bottom. level convolutional layer, the decoder contains multi-level deconvolutional layers that are progressively linked from bottom to top, the last convolutional layer of the encoder is forwardly linked with the first deconvolutional layer of the decoder, and the remaining convolutional layers of the encoder The product layer has a one-to-one skip connection with the remaining deconvolutional layers of the decoder, including the following:
[0042] A. Automatically cut out the...
PUM
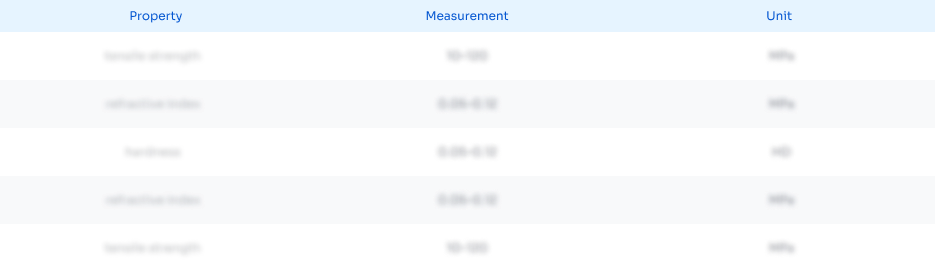
Abstract
Description
Claims
Application Information
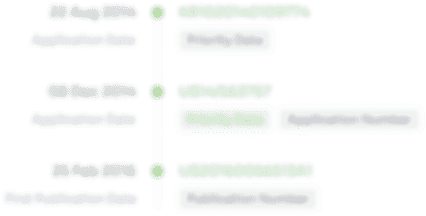
- R&D
- Intellectual Property
- Life Sciences
- Materials
- Tech Scout
- Unparalleled Data Quality
- Higher Quality Content
- 60% Fewer Hallucinations
Browse by: Latest US Patents, China's latest patents, Technical Efficacy Thesaurus, Application Domain, Technology Topic, Popular Technical Reports.
© 2025 PatSnap. All rights reserved.Legal|Privacy policy|Modern Slavery Act Transparency Statement|Sitemap|About US| Contact US: help@patsnap.com