Night image enhancement method and system based on cyclic generative adversarial residual network and QTP loss item
An image enhancement and network technology, applied in the field of computer vision, can solve problems such as insufficient night enhancement effect, poor image blur quality, and loss of semantic information
- Summary
- Abstract
- Description
- Claims
- Application Information
AI Technical Summary
Problems solved by technology
Method used
Image
Examples
Embodiment Construction
[0056] In conjunction with the following specific embodiments and accompanying drawings, the invention will be further described in detail. The process, conditions, experimental methods, etc. for implementing the present invention, except for the content specifically mentioned below, are common knowledge and common knowledge in this field, and the present invention has no special limitation content.
[0057] The present invention comprises the following concrete steps:
[0058] Step 1: Obtain the night scene image and the corresponding daytime scene image, divide the data set, and divide the data set into a test set and a training set according to the ratio of the number of test sets to the number of training sets at 1:30. Then perform data pre-operations: cropping the image, scaling the size of the image (the size of the cropped image and the size of the zoomed image need to be a multiple of 4, the specific size depends on the actual graphics card memory), image rotation and ...
PUM
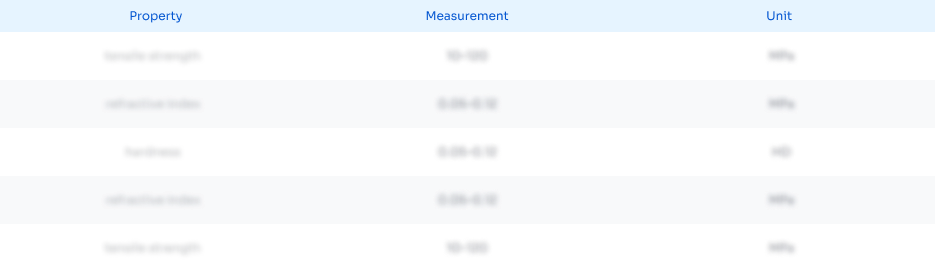
Abstract
Description
Claims
Application Information
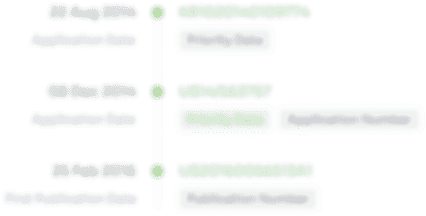
- R&D Engineer
- R&D Manager
- IP Professional
- Industry Leading Data Capabilities
- Powerful AI technology
- Patent DNA Extraction
Browse by: Latest US Patents, China's latest patents, Technical Efficacy Thesaurus, Application Domain, Technology Topic, Popular Technical Reports.
© 2024 PatSnap. All rights reserved.Legal|Privacy policy|Modern Slavery Act Transparency Statement|Sitemap|About US| Contact US: help@patsnap.com