Method for predicting antibacterial peptides of lactic acid bacteria based on graph neural network
A neural network and prediction method technology, applied in the field of identification of biological antimicrobial peptides, can solve problems such as time-consuming, accuracy rate improvement, and difficulty in grasping antimicrobial peptides, and achieve the effect of batch identification and identification accuracy index improvement
- Summary
- Abstract
- Description
- Claims
- Application Information
AI Technical Summary
Problems solved by technology
Method used
Image
Examples
Embodiment Construction
[0023] The technical solutions of the present invention will be further described below in conjunction with the accompanying drawings and embodiments.
[0024] As shown in the figure, a lactic acid bacteria antimicrobial peptide prediction method based on graph neural network is mainly divided into four aspects: data collection, model establishment, model tuning, and model prediction.
[0025] Specifically, it can be subdivided into the following steps:
[0026] S1. Data collection, establishment of positive samples and negative samples
[0027] Positive samples are the collection of lactic acid bacteria antimicrobial peptide sequences isolated from the comprehensive and special antimicrobial peptide database obtained from the survey, and negative samples are protein sequence collections that meet the length requirement of 5-255. The sample set is established based on the positive samples and negative samples.
[0028] Such as figure 2 As shown, lactic acid bacteria antibac...
PUM
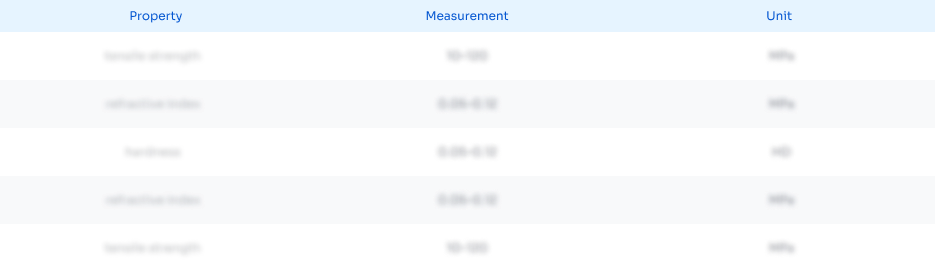
Abstract
Description
Claims
Application Information
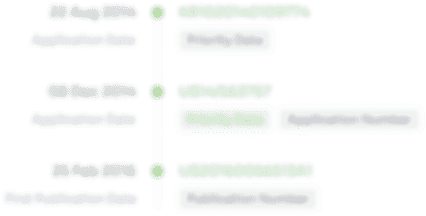
- R&D Engineer
- R&D Manager
- IP Professional
- Industry Leading Data Capabilities
- Powerful AI technology
- Patent DNA Extraction
Browse by: Latest US Patents, China's latest patents, Technical Efficacy Thesaurus, Application Domain, Technology Topic, Popular Technical Reports.
© 2024 PatSnap. All rights reserved.Legal|Privacy policy|Modern Slavery Act Transparency Statement|Sitemap|About US| Contact US: help@patsnap.com