Steganography detection feature selection method based on MMD residual error
A technology of steganography detection and feature selection, applied in instruments, character and pattern recognition, electrical components, etc., it can solve the problem of low dimensionality reduction effect, and achieve the effect of reducing feature dimensionality and maintaining detection performance.
- Summary
- Abstract
- Description
- Claims
- Application Information
AI Technical Summary
Problems solved by technology
Method used
Image
Examples
Embodiment Construction
[0030]In order to make the purpose, technical solutions and advantages of the present invention clearer, the technical solutions in the embodiments of the present invention will be clearly described below in conjunction with the accompanying drawings in the embodiments of the present invention. Obviously, the described embodiments are part of the present invention Examples, not all examples. Based on the embodiments of the present invention, all other embodiments obtained by persons of ordinary skill in the art without making creative efforts belong to the protection scope of the present invention.
[0031] MMD: Maximum Mean Discrepancy, the maximum mean difference.
[0032] Such as figure 1 As shown, the embodiment of the present invention provides a method of feature selection for steganographic detection based on MMD residuals, comprising the following steps:
[0033] S101: Disassemble the high-dimensional Rich Model steganographic detection features into several Rich Mod...
PUM
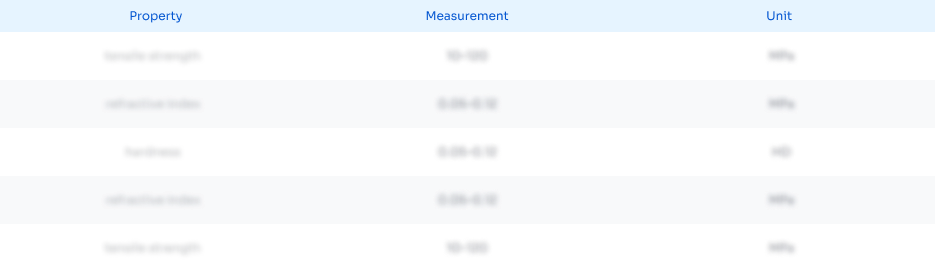
Abstract
Description
Claims
Application Information
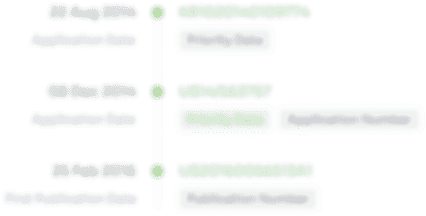
- R&D Engineer
- R&D Manager
- IP Professional
- Industry Leading Data Capabilities
- Powerful AI technology
- Patent DNA Extraction
Browse by: Latest US Patents, China's latest patents, Technical Efficacy Thesaurus, Application Domain, Technology Topic, Popular Technical Reports.
© 2024 PatSnap. All rights reserved.Legal|Privacy policy|Modern Slavery Act Transparency Statement|Sitemap|About US| Contact US: help@patsnap.com