Video Semantic Segmentation Using Multi-Frequency Dynamic Atrous Convolution
A semantic segmentation and video technology, applied in the field of computer vision, can solve the problems of poor fault tolerance, large memory usage, bloated models, etc., to reduce computational complexity, improve processing efficiency, and improve segmentation speed.
- Summary
- Abstract
- Description
- Claims
- Application Information
AI Technical Summary
Problems solved by technology
Method used
Image
Examples
Embodiment Construction
[0041] The present invention will be further described below with reference to the accompanying drawings.
[0042] like figure 1 , using the video semantic segmentation method of multi-frequency dynamic hole convolution, firstly sample the given video and input it into the encoder composed of convolutional neural network to obtain the shallow visual feature map of the video frame; The feature frequency separation module composed of , Gaussian filter, and inverse Fourier transform separates the multi-frequency feature map from the shallow visual feature map; and then uses the dynamic feature map composed of a weight calculator and multiple parallel hole convolution kernels. The atrous convolution processes the multi-frequency feature maps at different depths to obtain the multi-frequency high-level semantic feature maps; finally, the multi-frequency high-level semantic feature maps are spliced and input to the decoder for up-sampling to obtain the semantic segmentation result...
PUM
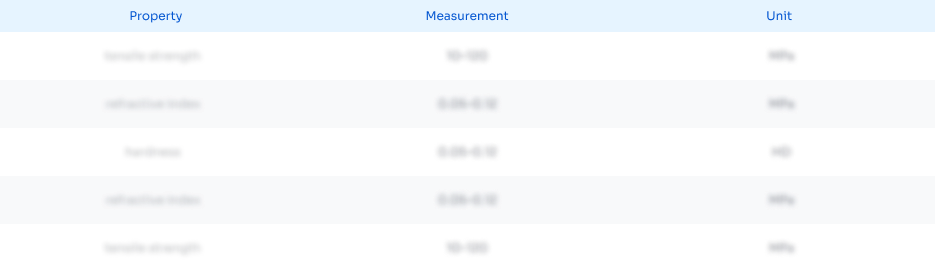
Abstract
Description
Claims
Application Information
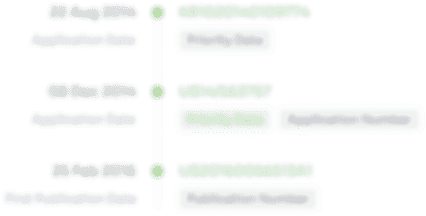
- R&D Engineer
- R&D Manager
- IP Professional
- Industry Leading Data Capabilities
- Powerful AI technology
- Patent DNA Extraction
Browse by: Latest US Patents, China's latest patents, Technical Efficacy Thesaurus, Application Domain, Technology Topic, Popular Technical Reports.
© 2024 PatSnap. All rights reserved.Legal|Privacy policy|Modern Slavery Act Transparency Statement|Sitemap|About US| Contact US: help@patsnap.com