Data enhancement-based out-of-distribution abnormal sample detection method
A technology of abnormal samples and detection methods, applied in the direction of instruments, character and pattern recognition, computing models, etc., can solve problems such as model prediction uncertainty, model security, etc.
- Summary
- Abstract
- Description
- Claims
- Application Information
AI Technical Summary
Problems solved by technology
Method used
Image
Examples
Embodiment Construction
[0023] In order to better understand the purpose, structure and function of the present invention, a method for detecting out-of-distribution abnormal samples based on data enhancement of the present invention will be further described in detail below in conjunction with the accompanying drawings.
[0024] Such as figure 1 Shown, the present invention comprises the following steps:
[0025] Step 1, feature extraction, use the automatic encoder algorithm to extract the feature vector of the sample picture in the input distribution.
[0026] Step 1.1. Map the input sample picture to the feature space Z through the encoder to obtain the abstract feature z;
[0027] Step 1.2, map the abstract feature z back to the input space X to obtain reconstructed samples;
[0028] Step 1.3. Simultaneously optimize the encoder and decoder by minimizing the reconstruction error to obtain the optimal model parameters;
[0029] Step 1.4, output the abstract feature representation z* for the sa...
PUM
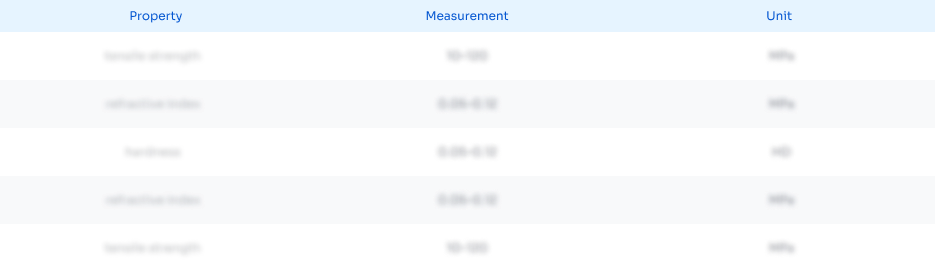
Abstract
Description
Claims
Application Information
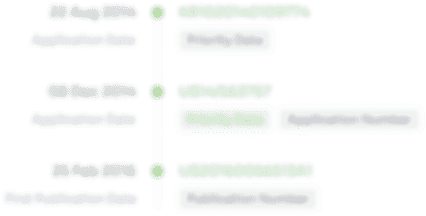
- R&D Engineer
- R&D Manager
- IP Professional
- Industry Leading Data Capabilities
- Powerful AI technology
- Patent DNA Extraction
Browse by: Latest US Patents, China's latest patents, Technical Efficacy Thesaurus, Application Domain, Technology Topic, Popular Technical Reports.
© 2024 PatSnap. All rights reserved.Legal|Privacy policy|Modern Slavery Act Transparency Statement|Sitemap|About US| Contact US: help@patsnap.com