Liver tumor recognition method based on self-supervised dense convolutional neural network
A convolutional neural network and recognition method technology, applied in the field of liver tumor recognition based on self-supervised dense convolutional neural network, can solve the problems of insignificant early symptoms of HCC and insufficient training sample data, so as to reduce the amount of calculation and improve the calculation. Efficiency and improved accuracy
- Summary
- Abstract
- Description
- Claims
- Application Information
AI Technical Summary
Problems solved by technology
Method used
Image
Examples
Embodiment Construction
[0038] The present invention will be further described in detail below in conjunction with the accompanying drawings, which are explanations rather than limitations of the present invention.
[0039] refer to figure 1 , a liver tumor identification method based on a self-supervised dense convolutional neural network, including the following steps:
[0040] Step 1. According to the patient's magnetic resonance image, slice from two cross directions to obtain the liver slice dataset {A};
[0041] Specifically, a liver magnetic resonance imaging (MRI) technique is used to acquire medical images. Slices are made from two intersecting directions, assuming that the conventional medical slice direction is the main direction to cut M slices, and a direction perpendicular to the main direction is selected as the auxiliary direction to cut N slices, and a total of M+N slices can be obtained from one patient , where M and N are assumed to be 10, and the MRI slice images of multiple pat...
PUM
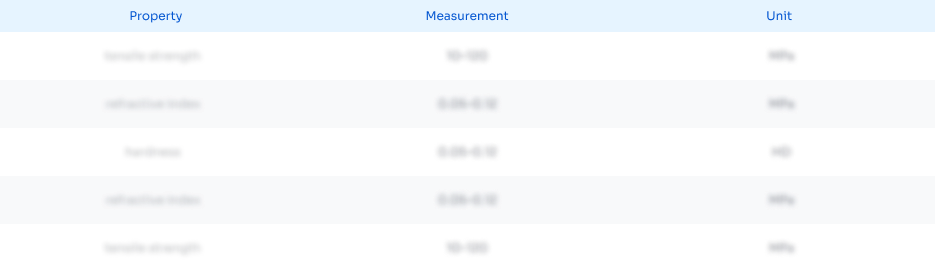
Abstract
Description
Claims
Application Information
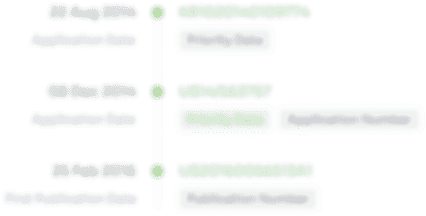
- R&D Engineer
- R&D Manager
- IP Professional
- Industry Leading Data Capabilities
- Powerful AI technology
- Patent DNA Extraction
Browse by: Latest US Patents, China's latest patents, Technical Efficacy Thesaurus, Application Domain, Technology Topic, Popular Technical Reports.
© 2024 PatSnap. All rights reserved.Legal|Privacy policy|Modern Slavery Act Transparency Statement|Sitemap|About US| Contact US: help@patsnap.com