Seismic positioning method and equipment based on fusion of machine learning and dynamic computing
A technology of machine learning and positioning methods, applied in the field of earthquake positioning, can solve problems such as limited historical data and inaccurate source locations
- Summary
- Abstract
- Description
- Claims
- Application Information
AI Technical Summary
Problems solved by technology
Method used
Image
Examples
Embodiment 1
[0057] This embodiment implements an earthquake location method based on the fusion of machine learning and dynamic calculation, such as figure 1 shown, including the following steps:
[0058] S1. Acquire multi-source data, and perform regularization processing on the multi-source data;
[0059] S2. Perform short-time Fourier transform on the regularized data to obtain a time-frequency image as a sample, generate label data for each sample according to the three-dimensional Gaussian distribution according to the source position, and use the cross-validation method to generate a training set and a test set;
[0060] S3. Perform full convolutional neural network model training based on the training set, and evaluate the accuracy of the trained model based on the test set to obtain a trained model;
[0061] S4. Input the real-time monitoring data into the trained model to preliminarily estimate the source position;
[0062] S5. Correct the preliminary estimated hypocenter posit...
Embodiment 2
[0086] This embodiment implements an earthquake location method based on machine learning and dynamic calculation fusion, including the following steps:
[0087] Obtain multi-source data, and perform regularization processing on the multi-source data;
[0088] Perform short-time Fourier transform on the regularized data to obtain time-frequency images as samples, generate label data for each sample according to the three-dimensional Gaussian distribution according to the source position, and use cross-validation method to generate training set and test set;
[0089] The full convolutional neural network model is trained based on the training set, and the accuracy of the trained model is evaluated based on the test set to obtain the trained model;
[0090] Input the real-time monitoring data into the trained model to preliminarily estimate the source location;
[0091] The initial estimation of the hypocentral position is corrected by the dynamic calculation model.
[0092] T...
PUM
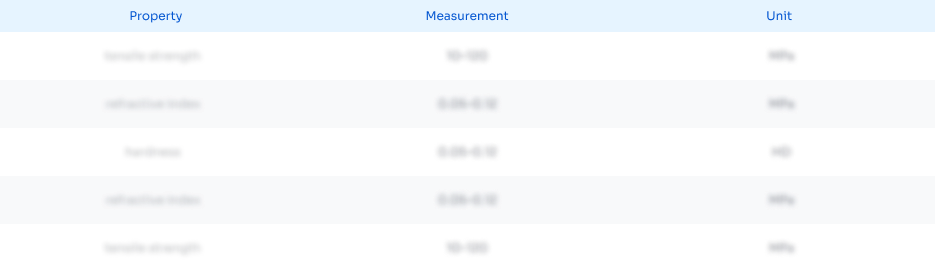
Abstract
Description
Claims
Application Information
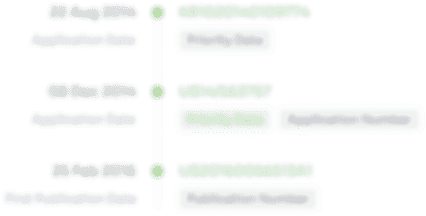
- R&D Engineer
- R&D Manager
- IP Professional
- Industry Leading Data Capabilities
- Powerful AI technology
- Patent DNA Extraction
Browse by: Latest US Patents, China's latest patents, Technical Efficacy Thesaurus, Application Domain, Technology Topic, Popular Technical Reports.
© 2024 PatSnap. All rights reserved.Legal|Privacy policy|Modern Slavery Act Transparency Statement|Sitemap|About US| Contact US: help@patsnap.com