Efficient medical image marking and learning system
A medical imaging and learning system technology, applied in the field of efficient medical image labeling and learning systems, can solve the problems of unbalanced categories, affecting the use effect, time-consuming and labor-intensive, etc., and achieve the effect of reducing dependence and optimizing the learning effect.
- Summary
- Abstract
- Description
- Claims
- Application Information
AI Technical Summary
Problems solved by technology
Method used
Image
Examples
Embodiment Construction
[0028] The following is a preferred embodiment of the present invention and the technical solution of the present invention is further described in conjunction with the accompanying drawings, but the present invention is not limited to this embodiment.
[0029]The present invention proposes an efficient medical image labeling and learning system, which mainly includes introducing unsupervised contrastive learning in the pre-training process to utilize a large amount of unlabeled data; in the following formal training stage, introducing active learning in unlabeled data Select difficult examples for labeling and training to reduce the need for labeling, and achieve efficient use of limited data through comparative learning, and build a data labeling platform to complete the cycle of labeling the extracted key samples-training-re-extracting samples The training process leads to an efficient and cost-effective deep learning network model on different types of medical images includ...
PUM
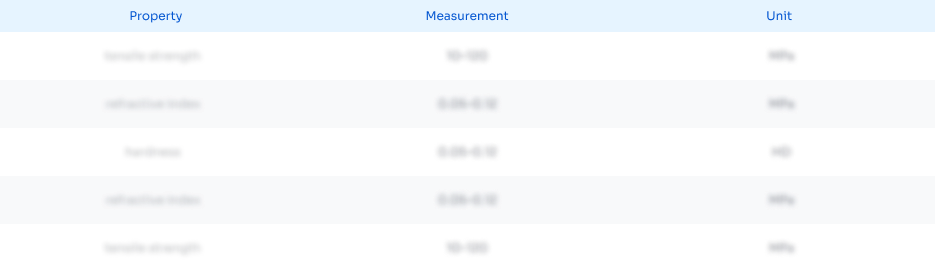
Abstract
Description
Claims
Application Information
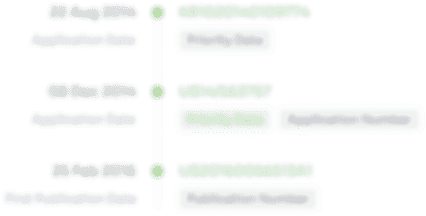
- R&D Engineer
- R&D Manager
- IP Professional
- Industry Leading Data Capabilities
- Powerful AI technology
- Patent DNA Extraction
Browse by: Latest US Patents, China's latest patents, Technical Efficacy Thesaurus, Application Domain, Technology Topic.
© 2024 PatSnap. All rights reserved.Legal|Privacy policy|Modern Slavery Act Transparency Statement|Sitemap