A land and water segmentation method for unsupervised domain adaptation of remote sensing images
A technology of remote sensing image, water and land segmentation
- Summary
- Abstract
- Description
- Claims
- Application Information
AI Technical Summary
Problems solved by technology
Method used
Image
Examples
Embodiment 1
[0024] See attached figure 1 , the present invention uses the remote sensing band data formed by Landsat satellites to synthesize four-channel images through linear stretching, so that the image style is similar to that of the public labeled data set GID. Then, a generative confrontation training convolutional neural network is designed based on AdaptSegnet, and the supervised GID dataset and the unsupervised landsat8 dataset are mapped to the same feature space for segmentation. At the same time, the NDWI value of the image is linked to the feature space, and the detailed information of the image is preserved. During training, two data sets are alternately passed in. For the GID data set, the generation network G and the discriminant network D in the network model are optimized, and for the landsat8 data set, the discriminant network D in the network model is optimized. Finally, the input Landsat satellite remote sensing images can be output to predict the land and water seg...
PUM
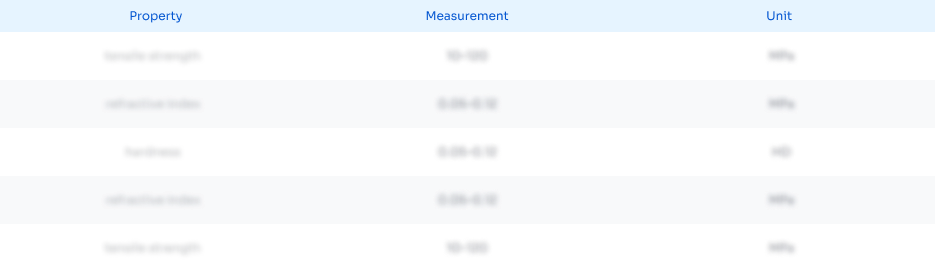
Abstract
Description
Claims
Application Information
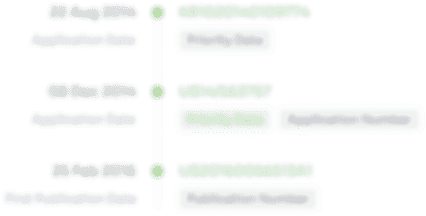
- R&D Engineer
- R&D Manager
- IP Professional
- Industry Leading Data Capabilities
- Powerful AI technology
- Patent DNA Extraction
Browse by: Latest US Patents, China's latest patents, Technical Efficacy Thesaurus, Application Domain, Technology Topic, Popular Technical Reports.
© 2024 PatSnap. All rights reserved.Legal|Privacy policy|Modern Slavery Act Transparency Statement|Sitemap|About US| Contact US: help@patsnap.com