Patch-mounting anomaly detection method fusing CMA-ES algorithm and sequential extreme learning machine
An extreme learning machine, anomaly detection technology, applied in the field of computer vision, can solve the problems of the model cannot be modified, optimized, limited and robust, weak generalization ability, etc., to achieve less learning parameters, good global performance, improve Detecting the effect of speed
- Summary
- Abstract
- Description
- Claims
- Application Information
AI Technical Summary
Problems solved by technology
Method used
Image
Examples
Embodiment Construction
[0069] In this example, if figure 1 As shown, a post-patch anomaly detection method that combines CMA-ES (covariance matrix adaptive evolution strategy) algorithm and sequential extreme learning machine is carried out as follows:
[0070] Step 1. Obtain the gray histograms of N normal soldered PCBs from the normal soldered PCB database to form a sample training set;
[0071] Step 2, image enhancement is performed on the training set to obtain an enhanced training set;
[0072] Step 2.1, convert the N grayscale histograms in the training set into a uniform distribution map, that is, the number of pixels of the image above each grayscale level is the same, so as to achieve the effect of increasing the dynamic value range. Set the abscissa as the image grayscale vertical The coordinates are the frequency at which grayscale pixels appear in the image, and the grayscale range is [l 1 , l 2 ] then its grayscale histogram function is:
[0073] h(r k ) = n k ,k∈[0,L-1] (1)
[0...
PUM
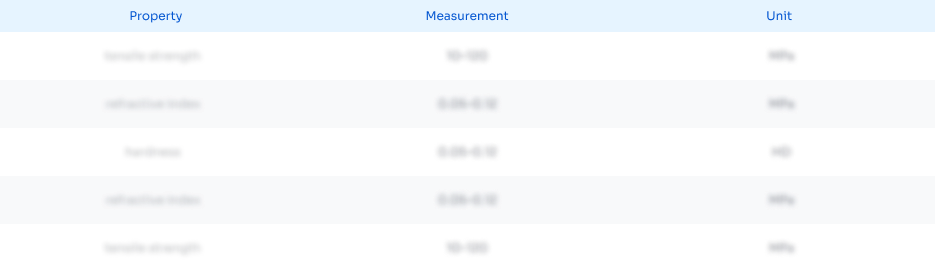
Abstract
Description
Claims
Application Information
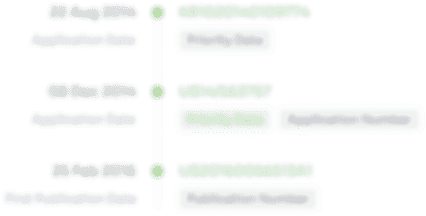
- R&D Engineer
- R&D Manager
- IP Professional
- Industry Leading Data Capabilities
- Powerful AI technology
- Patent DNA Extraction
Browse by: Latest US Patents, China's latest patents, Technical Efficacy Thesaurus, Application Domain, Technology Topic, Popular Technical Reports.
© 2024 PatSnap. All rights reserved.Legal|Privacy policy|Modern Slavery Act Transparency Statement|Sitemap|About US| Contact US: help@patsnap.com