A Method for Knowledge Mining and Parallel Processing of Massive Traffic Data
A traffic data and knowledge mining technology, which is applied in the field of traffic big data, can solve problems such as inability to predict online increments and long time-consuming large-scale neural networks, and achieve the effect of reducing the time spent on communication, reducing the time-consuming communication, and reducing the number of parameters
- Summary
- Abstract
- Description
- Claims
- Application Information
AI Technical Summary
Problems solved by technology
Method used
Image
Examples
Embodiment 1
[0045] Embodiment 1: It is divided into a model training process and an actual prediction process.
[0046] (1) Model training process
[0047] Step 1: Construction of Flight Track Prediction Model
[0048] The flight track prediction model is designed with LSTM, and the specific parameters of the model are designed as follows: the number of input layer nodes is set to 6, the number of output layer nodes is set to 1, the prediction time step is designed to be 6, the number of hidden layers is set to 1, and the number of hidden layers is set to 1. The number of nodes is set to 60, the number of training rounds is set to 50, the activation function uses ReLU, the loss function uses the cross-entropy function, and the optimization method of the loss function uses the stochastic gradient descent method.
[0049] Step 2: Build the input data
[0050] Obtain the historical ADS-B data of multiple flights, extract flight data from the historical ADS-B data in six fields including fl...
PUM
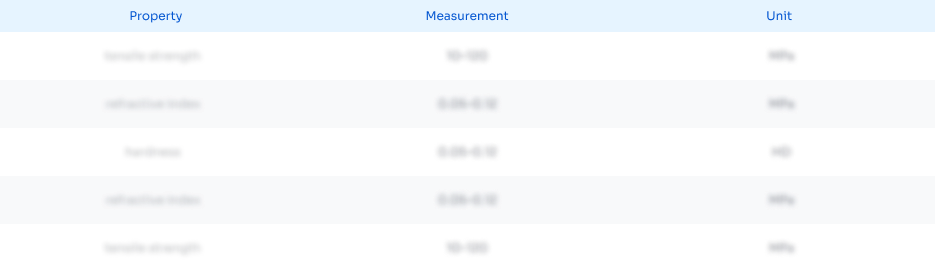
Abstract
Description
Claims
Application Information
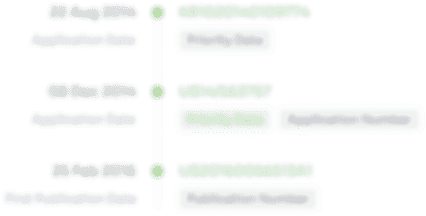
- R&D Engineer
- R&D Manager
- IP Professional
- Industry Leading Data Capabilities
- Powerful AI technology
- Patent DNA Extraction
Browse by: Latest US Patents, China's latest patents, Technical Efficacy Thesaurus, Application Domain, Technology Topic, Popular Technical Reports.
© 2024 PatSnap. All rights reserved.Legal|Privacy policy|Modern Slavery Act Transparency Statement|Sitemap|About US| Contact US: help@patsnap.com