Video super-resolution method based on multi-frame attention mechanism progressive fusion
A technology of super-resolution and attention, applied in the direction of instruments, biological neural network models, graphics and image conversion, etc., can solve the problems of difficult estimation of accurate flow information, sensitivity of deformable convolution input, and affecting the quality of video reconstruction, etc. Achieve the effects of reducing fusion difficulty, accelerating convergence, and improving super-resolution efficiency
- Summary
- Abstract
- Description
- Claims
- Application Information
AI Technical Summary
Problems solved by technology
Method used
Image
Examples
Embodiment
[0078] This embodiment provides a video super-resolution method based on multi-frame attention mechanism progressive fusion, such as figure 1 shown, including the following steps:
[0079] S1. Video decoding: use the ffmpeg tool to extract frames from the video data set and save them as pictures to generate a training set.
[0080] Here, the video dataset contains high-resolution videos and low-resolution videos with the same video content. High-resolution videos refer to videos that reach the target resolution, and low-resolution videos refer to videos that are lower than the target resolution.
[0081] All the frames of the high and low resolution videos are reserved, and each low resolution video image has a corresponding high resolution video image to form the initial training set; the initial training set has N pairs of images: {(x 1L ,x 1H ),(x 2L ,x 2H ),…,(x NL ,x NH)}, where x NL Represents the low-resolution video image in the Nth pair of images; x NH Represe...
PUM
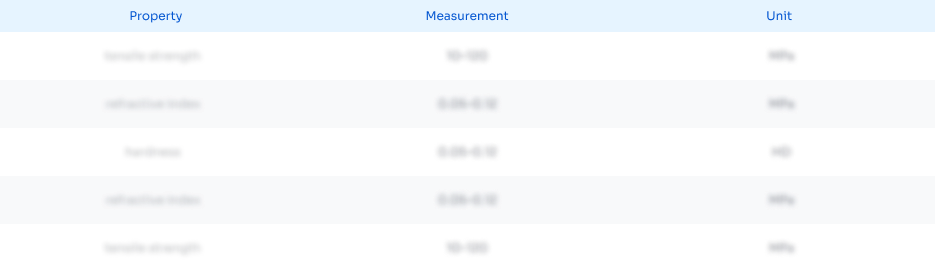
Abstract
Description
Claims
Application Information
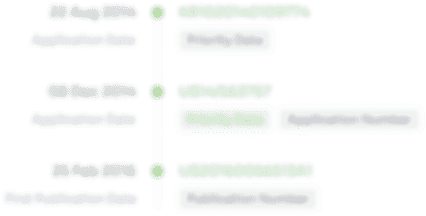
- R&D Engineer
- R&D Manager
- IP Professional
- Industry Leading Data Capabilities
- Powerful AI technology
- Patent DNA Extraction
Browse by: Latest US Patents, China's latest patents, Technical Efficacy Thesaurus, Application Domain, Technology Topic, Popular Technical Reports.
© 2024 PatSnap. All rights reserved.Legal|Privacy policy|Modern Slavery Act Transparency Statement|Sitemap|About US| Contact US: help@patsnap.com