Method and system for detecting quality of simulated user experience in dialogue strategy learning
A technology for simulating users and policy learning, applied in the field of machine learning, can solve problems such as hyper-parameter selection is highly sensitive, unstable, and dialogue learning performance constraints, and achieve the effect of effectively controlling the quality of simulation experience and the quality of relaxation
- Summary
- Abstract
- Description
- Claims
- Application Information
AI Technical Summary
Problems solved by technology
Method used
Image
Examples
Embodiment 1
[0029] Such as figure 1 As shown, this scheme proposes a method for detecting the quality of simulated user experience in dialogue policy learning. Dialogue strategy learning. The dialogue policy learning of the dialogue policy model mainly includes two parts: direct reinforcement learning and indirect reinforcement learning (also called planning). Direct reinforcement learning, using Deep Q-Network (DQN) to improve the dialogue policy based on real experience, the dialogue policy model interacts with the user User, in each step, the dialogue policy model maximizes the value function Q according to the observed dialogue state s, Select the action a to perform. Then, the dialog policy model receives the reward r, the real user's action a r u , and update the current state to s’, and then the real experience (s, a, r, a r u , t) is stored in the real user experience database, and t is used to indicate whether the dialogue is terminated.
[0030] Maximize the value functi...
Embodiment 2
[0045] This embodiment is similar to Embodiment 1, the difference is that this embodiment considers that in the initial stage, there are only limited actions (behaviors) in the lexicon world-dict, so the length of the lexicon same-dict is also very small, in order to predict For the thermal world model, preferably when the length of the lexicon same-dict is less than the constant C, the simulation experience is regarded as qualified. The constant C is determined by those skilled in the art according to specific conditions, and is not limited here.
[0046] At this time, only when the length of the thesaurus same-dict reaches a certain value, that is, when it is greater than or equal to the constant C, the variable KL defined in advance is passed. pre Track the KL divergence between thesaurus real-dict and thesaurus world-dict for similarity measurement.
Embodiment 3
[0048] This embodiment provides a system for detecting the quality of simulated user experience in dialogue strategy learning, which is used to implement the method in Embodiment 1 or Embodiment 2, including a system connected to the world model, the real user experience library, and the dialogue strategy model. A quality detector, and the quality detector includes a KL divergence detector, and the KL divergence detector is used to detect the quality of the simulated experience generated by the world model according to the real experience generated by the real user.
[0049] Specifically, the quality detector includes a thesaurus real-dict for storing real experience, a thesaurus world-dict for storing simulated experience, and a primary key for saving the intersection of the thesaurus real-dict and thesaurus world-dict in two Thesaurus same-dict of frequency values in a thesaurus.
PUM
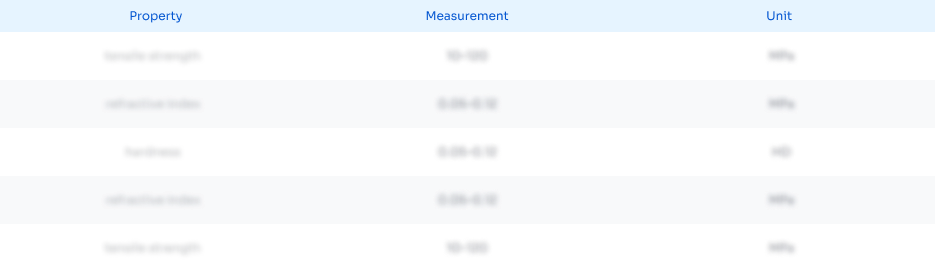
Abstract
Description
Claims
Application Information
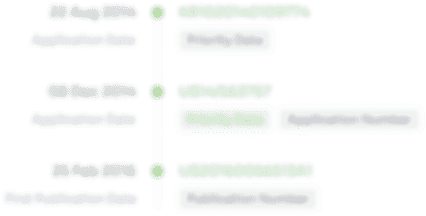
- R&D Engineer
- R&D Manager
- IP Professional
- Industry Leading Data Capabilities
- Powerful AI technology
- Patent DNA Extraction
Browse by: Latest US Patents, China's latest patents, Technical Efficacy Thesaurus, Application Domain, Technology Topic, Popular Technical Reports.
© 2024 PatSnap. All rights reserved.Legal|Privacy policy|Modern Slavery Act Transparency Statement|Sitemap|About US| Contact US: help@patsnap.com