Low-frequency seismic data reconstruction method based on multi-seismic-source convolutional neural network
A convolutional neural network and seismic data technology, applied in the field of low-frequency seismic data reconstruction, can solve the problems of ignoring the application of passive source seismic signals, unknown source wavelet and source position, weak signal energy, etc., reaching the level of instrument and signal processing Reduced requirements, reduced collection costs, and high accuracy
- Summary
- Abstract
- Description
- Claims
- Application Information
AI Technical Summary
Problems solved by technology
Method used
Image
Examples
Embodiment 1
[0040] This embodiment discloses a low-frequency seismic data reconstruction method based on a multi-source convolutional neural network, figure 1 For a specific work flow chart, the following steps are included:
[0041] a. Seismic data preprocessing: perform static correction processing on the original active source seismic data to correct the influence of the undulating surface on the reflection coaxial axis; perform denoising processing on the data to remove microseismic, background noise and other random noise; remove interference waves, Including sound waves, surface waves, industrial electrical interference, ghost reflections, multiple reflections, side waves, bottom waves, reverberation and ringing, etc. Finally, high-quality observed seismic data are obtained;
[0042] b. Process the processed active source data and the original passive source data separately: Passive source seismic data is low-pass filtered through a Butterworth low-pass filter to obtain passive sou...
Embodiment 2
[0054] The following is a detailed introduction to Embodiment 2 of a seismic data reconstruction method based on a multi-source convolutional neural network provided by this application. Embodiment 2 is implemented based on the foregoing Embodiment 1, and is carried out to a certain extent on the basis of Embodiment 1. expansion.
[0055] We demonstrate the success of the entire convolutional neural network on the Marmousi velocity model. Figure 5(a) is the simulated passive source seismic data obtained by cross-correlation method, and Figure 5(b) is the passive source low-frequency seismic data obtained after low-pass filtering. Figure 6(a) and (b) show the active source seismic data obtained from forward modeling and their corresponding low-frequency data. The low-frequency part of the simulated passive source seismic data is input into a pre-trained convolutional neural network to perform low-frequency reconstruction on the active source seismic data. Finally, the obtaine...
PUM
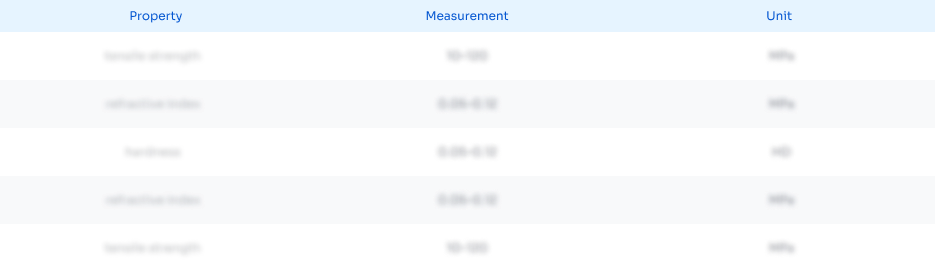
Abstract
Description
Claims
Application Information
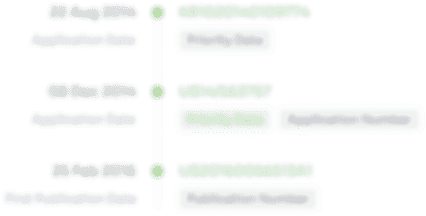
- R&D Engineer
- R&D Manager
- IP Professional
- Industry Leading Data Capabilities
- Powerful AI technology
- Patent DNA Extraction
Browse by: Latest US Patents, China's latest patents, Technical Efficacy Thesaurus, Application Domain, Technology Topic, Popular Technical Reports.
© 2024 PatSnap. All rights reserved.Legal|Privacy policy|Modern Slavery Act Transparency Statement|Sitemap|About US| Contact US: help@patsnap.com