An Online Fault Diagnosis Method for Rolling Bearings under Variable Load Based on Transfer Learning
A rolling bearing and fault diagnosis technology, which is applied in the field of online fault diagnosis of rolling bearings under variable load of transfer learning, can solve the problems such as the inability to effectively guarantee the modeling efficiency and accuracy of online fault diagnosis of rolling bearings under variable load, so as to solve the problem of online fault diagnosis, The effect of high recognition accuracy and good generalization
- Summary
- Abstract
- Description
- Claims
- Application Information
AI Technical Summary
Problems solved by technology
Method used
Image
Examples
specific Embodiment 1
[0086] In order to verify the validity and accuracy of the method of the present invention, the bearing data set of Case Western Reserve University in the United States is used for experimental verification. [23] . Bearing test benches such as Figure 4 As shown, these data were collected by the accelerometer under 4 load conditions and the sampling frequency was 12kHz.
[0087] Load types include 0hp, 1hp, 2hp and 3hp, and the motor speed varies between 1730rpm and 1797rpm depending on the load. Under each load, there are 3 fault damage degrees including normal state (N) and inner ring fault (IR), outer ring fault (OR), and rolling element fault (B). The damage diameters are 0.1778mm, 0.3556mm, 0.5334mm respectively mm, there is no damage to the rolling bearing under normal conditions, so there is no fault location and degree of fault damage. The normal state of the rolling bearing, inner ring, outer ring, rolling element failure and 10 states of different failure damage l...
specific Embodiment 2
[0115] In order to better verify the efficiency of the method of the present invention, the time consumption and accuracy of the three methods are compared, wherein the settings of the online data set are consistent with Table 5, and the experimental results are shown in Table 7.
[0116] Table 7 Time consumption and accuracy comparison of three methods
[0117]
[0118] In the experiment, Method 1 and Method 2 use offline training methods, that is, the model is retrained each time to process the newly added online data; Method 3 is an online training method, which only needs to update the model for the newly added online data. Method 1 is the combination of CNN and Softmax for back-propagation optimization training CNN model; Method 2 is to extract features from the fault data with the CNN model trained in Method 1, and then input SVM for fault classification; Method 3 is the CNN proposed by the present invention. - ISVM rolling bearing multi-state identification method. ...
specific Embodiment 3
[0120] In order to further prove that the method of the present invention has obvious advantages in the online fault diagnosis of rolling bearings under variable load, ISVM, OS-ELM are selected. [28] And the online learning method proposed in the literature [29] to conduct a comparative experimental study. Among them, ISVM and OS-ELM methods use the feature extraction method of literature [30] to extract 10 kinds of time-domain features and 4 kinds of frequency-domain features, and then input the extracted features into the diagnosis algorithm to complete the fault diagnosis. During the experiment, the same source domain and target domain datasets were used. The time comparison results are shown in Table 8, and the accuracy comparison results are shown in Table 8. Figure 11 shown.
[0121] Table 8 Time comparison with other methods
[0122]
[0123] It can be seen from Table 8 that the average online learning time of the method proposed in the present invention is 16.97s...
PUM
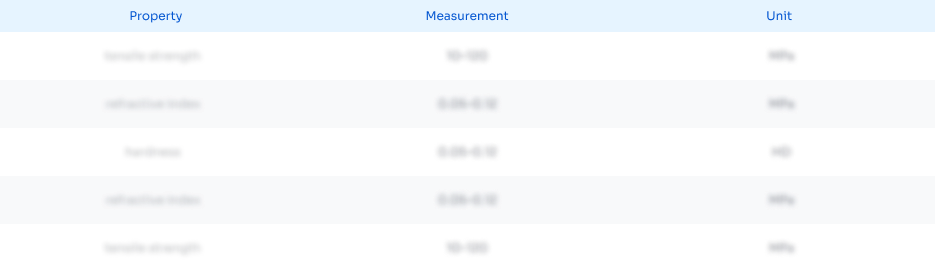
Abstract
Description
Claims
Application Information
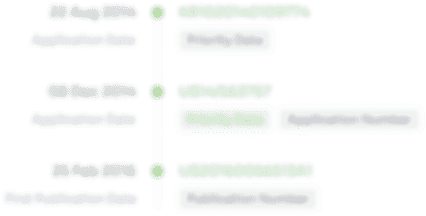
- R&D Engineer
- R&D Manager
- IP Professional
- Industry Leading Data Capabilities
- Powerful AI technology
- Patent DNA Extraction
Browse by: Latest US Patents, China's latest patents, Technical Efficacy Thesaurus, Application Domain, Technology Topic, Popular Technical Reports.
© 2024 PatSnap. All rights reserved.Legal|Privacy policy|Modern Slavery Act Transparency Statement|Sitemap|About US| Contact US: help@patsnap.com