Aerospace detonator production scheduling method based on deep reinforcement learning
A technology of intensive learning and production scheduling, applied in the direction of neural learning methods, based on specific mathematical models, instruments, etc., can solve the problems of losing scheduling rules, ignoring the value of experience records, lack of detonator trial assembly, and consideration of the impact of solidification quality, etc. Achieve the effects of improving training speed, high stability and adaptability, and improving the efficiency of detonator production and processing
- Summary
- Abstract
- Description
- Claims
- Application Information
AI Technical Summary
Problems solved by technology
Method used
Image
Examples
Embodiment
[0058] Embodiment: According to the characteristics of the production and processing of the detonator, the present invention considers the impact of the special production process of the detonator on the production and processing of the detonator, such as trial assembly, glue mixing, curing, and pressurized medicine, and is aimed at urgent tasks in the production process of the detonator. Machine failure, process change and other obvious and implicit disturbances provide a production scheduling method for aerospace initiators based on deep reinforcement learning to minimize the completion time and reduce equipment load, which can improve the adaptability and performance of production scheduling. Real-time, relieve the detonator production workshop's dependence on manual adjustment, and make the scheduling plan better adapt to the complex and dynamic actual production process.
[0059] In the present invention, the production and processing scheduling of the detonator is express...
PUM
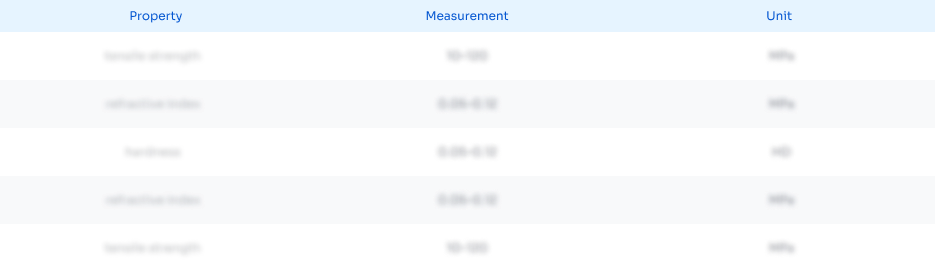
Abstract
Description
Claims
Application Information
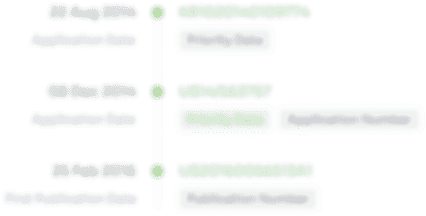
- R&D Engineer
- R&D Manager
- IP Professional
- Industry Leading Data Capabilities
- Powerful AI technology
- Patent DNA Extraction
Browse by: Latest US Patents, China's latest patents, Technical Efficacy Thesaurus, Application Domain, Technology Topic, Popular Technical Reports.
© 2024 PatSnap. All rights reserved.Legal|Privacy policy|Modern Slavery Act Transparency Statement|Sitemap|About US| Contact US: help@patsnap.com