High-resolution remote sensing image classification method based on residual network and transfer learning
A technology of remote sensing images and migration learning, which is applied in neural learning methods, biological neural network models, character and pattern recognition, etc. Difficult to be fully utilized and other issues to achieve the effect of improving scene classification, realizing scene classification, and wide coverage
- Summary
- Abstract
- Description
- Claims
- Application Information
AI Technical Summary
Problems solved by technology
Method used
Image
Examples
Embodiment Construction
[0048] In order to facilitate the understanding and implementation of the present invention by those of ordinary skill in the art, the present invention will be further described in detail below with reference to the accompanying drawings and embodiments. It should be understood that the embodiments described herein are only used to illustrate and explain the present invention, but not to limit it. this invention. The high-scoring remote sensing image classification method based on residual network and transfer learning of this application includes image feature extraction, transfer scene classification, and multi-scale result voting.
[0049] like figure 2 As shown, this application adopts the image feature extraction part and the classification process figure 1 As shown, the improved Resnet101 deep residual network performs feature extraction and pre-training on the source domain dataset; its basic steps include:
[0050] Step 1. Establish a source domain dataset and a te...
PUM
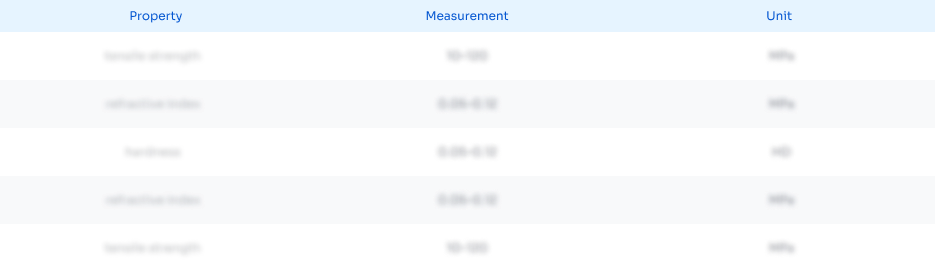
Abstract
Description
Claims
Application Information
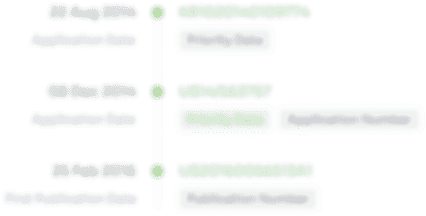
- R&D Engineer
- R&D Manager
- IP Professional
- Industry Leading Data Capabilities
- Powerful AI technology
- Patent DNA Extraction
Browse by: Latest US Patents, China's latest patents, Technical Efficacy Thesaurus, Application Domain, Technology Topic, Popular Technical Reports.
© 2024 PatSnap. All rights reserved.Legal|Privacy policy|Modern Slavery Act Transparency Statement|Sitemap|About US| Contact US: help@patsnap.com