Soil stress-strain relation determination method based on improved LSTM deep learning method
A soil stress-strain relationship technology, applied in neural learning methods, special data processing applications, instruments, etc., can solve problems such as inability to describe historical stress-strain effects, deviation of results, and failure to consider time-dependent characteristics of soil stress-strain behavior. , to achieve the effect of easy promotion, simple method and great application value
- Summary
- Abstract
- Description
- Claims
- Application Information
AI Technical Summary
Problems solved by technology
Method used
Image
Examples
Embodiment Construction
[0068] In order to make the purpose, technical solutions and advantages of the present invention clearer, the present invention will be further described in detail below in conjunction with the accompanying drawings.
[0069] The stress-strain behavior of a certain soil under different confining pressures conforms to the modified Cambridge model.
[0070] Such as figure 1 - figure 2 As shown, the present embodiment provides a method for determining the stress-strain relationship of soil based on long-short-term memory deep learning, and the method is realized through the following steps:
[0071] Step 1, preparing soil samples with different physical and mechanical parameters;
[0072] In this example, 29 numerical soil samples were established by using the numerical test method, and the value ranges of their physical and mechanical parameters are as follows: the compression index λ of the soil is 0.06, 0.09, 0.1, 0.12, and 0.15 respectively; the rebound index κ is 0.1*λ, ...
PUM
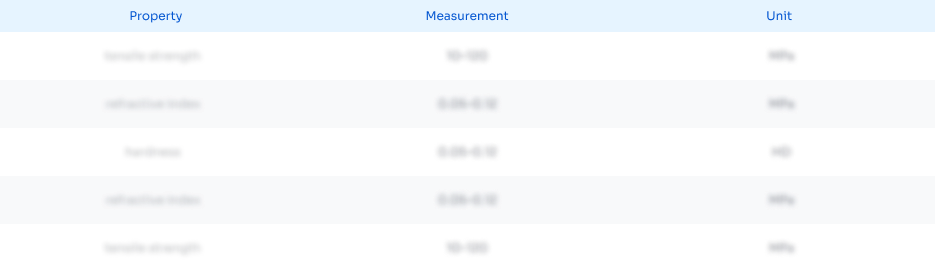
Abstract
Description
Claims
Application Information
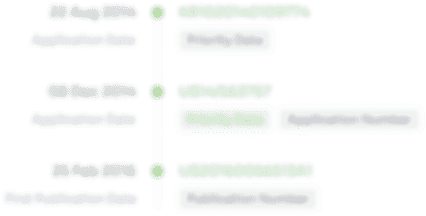
- R&D Engineer
- R&D Manager
- IP Professional
- Industry Leading Data Capabilities
- Powerful AI technology
- Patent DNA Extraction
Browse by: Latest US Patents, China's latest patents, Technical Efficacy Thesaurus, Application Domain, Technology Topic, Popular Technical Reports.
© 2024 PatSnap. All rights reserved.Legal|Privacy policy|Modern Slavery Act Transparency Statement|Sitemap|About US| Contact US: help@patsnap.com