Fine-grained image classification method based on multilayer focusing attention network
A focusing network, multi-layer focusing technology, applied in the field of computer vision, can solve the problems of similarity between classes, limited number of parts, limited classification accuracy, etc., to achieve the effect of improving robustness and reducing computational complexity
- Summary
- Abstract
- Description
- Claims
- Application Information
AI Technical Summary
Problems solved by technology
Method used
Image
Examples
Embodiment 1
[0031] Embodiment 1: as Figure 1-3 Shown, a kind of fine-grained image classification method based on multi-layer focused attention network, the concrete steps of described method are as follows:
[0032] Step1, the public data set CUB-200-2011 contains a total of 11788 images from 200 bird species, including 5994 training and verification images, and 5794 test images. Input the training image into the first-layer focus network, which is a single-layer focus convolution network combined with the convolution block attention feature module, which generates a feature and attention product matrix, and outputs the positioning area at the same time;
[0033] Step2, cropping and occlusion operation: the positioning area is obtained after the operation of Step1, and the cropping operation cuts the original image according to the positioning area to obtain a cropped image; the occlusion operation blocks the corresponding position of the original image according to the positioning area...
Embodiment 2
[0053] Example 2, such as Figure 1-3 As shown, a fine-grained image classification method based on a multi-layer focused attention network, this embodiment is the same as Embodiment 1, the difference is that in this embodiment, the public data set CUB-200-2011 (200 categories 11788 Bird images), FGVC-Aircraft (10,000 aircraft images of 100 categories) and Stanford Cars (16,185 car images of 196 categories) were evaluated respectively, and the Top-1 accuracy of 89.7%, 93.6%, and 95.1% were respectively obtained. The results obtained on the three fine-grained public datasets are compared with the current mainstream fine-grained image classification methods (VGG-19, ResNet-101, etc.) as shown in Table 2. The experimental results show that the classification accuracy of this method is higher than the current mainstream method.
[0054] Table 2 Comparison with the accuracy of the current method
[0055]
[0056]
[0057] The present invention proposes a fine-grained image ...
PUM
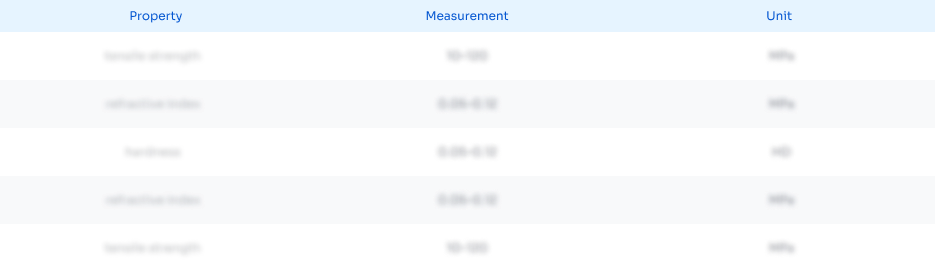
Abstract
Description
Claims
Application Information
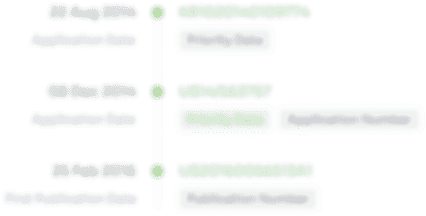
- R&D Engineer
- R&D Manager
- IP Professional
- Industry Leading Data Capabilities
- Powerful AI technology
- Patent DNA Extraction
Browse by: Latest US Patents, China's latest patents, Technical Efficacy Thesaurus, Application Domain, Technology Topic, Popular Technical Reports.
© 2024 PatSnap. All rights reserved.Legal|Privacy policy|Modern Slavery Act Transparency Statement|Sitemap|About US| Contact US: help@patsnap.com