A data-driven dynamic modeling method for deep neural network systems with large time delays
A deep neural network and dynamic modeling technology, applied in the field of dynamic modeling of deep neural network large time-delay systems, can solve the problems of slow update of weights and low efficiency.
- Summary
- Abstract
- Description
- Claims
- Application Information
AI Technical Summary
Problems solved by technology
Method used
Image
Examples
Embodiment Construction
[0019] The specific embodiments of the present invention are described below with reference to the accompanying drawings, so that those skilled in the art can better understand the present invention. It should be noted that, in the following description, when the detailed description of known functions and designs may dilute the main content of the present invention, these descriptions will be omitted here.
[0020] When the large delay system is a multi-input system, the mechanism analysis is used to analyze the multiple input data, and the relevant input data that affects the output of the system are preselected. However, considering the influence of various factors such as system operating conditions and external interference, the adaptive LASSO algorithm is used to re-screen the pre-selected input data.
[0021] The adaptive LASSO algorithm is as follows:
[0022]
[0023] in γ>0. It can be seen from the above formula that λ as the weight value of the penalty item o...
PUM
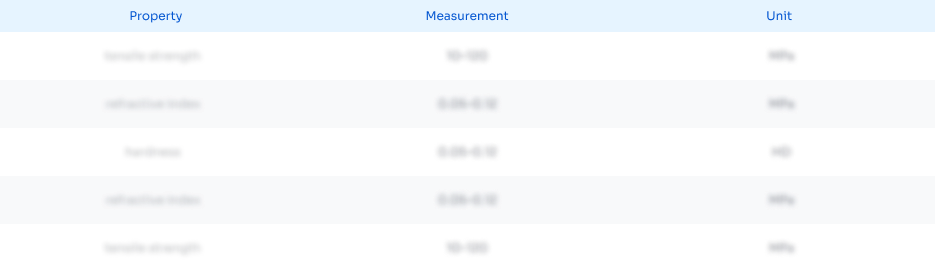
Abstract
Description
Claims
Application Information
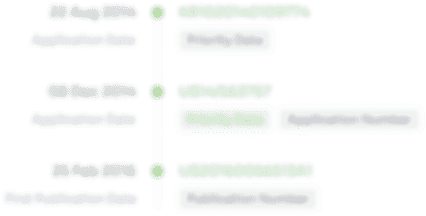
- Generate Ideas
- Intellectual Property
- Life Sciences
- Materials
- Tech Scout
- Unparalleled Data Quality
- Higher Quality Content
- 60% Fewer Hallucinations
Browse by: Latest US Patents, China's latest patents, Technical Efficacy Thesaurus, Application Domain, Technology Topic, Popular Technical Reports.
© 2025 PatSnap. All rights reserved.Legal|Privacy policy|Modern Slavery Act Transparency Statement|Sitemap|About US| Contact US: help@patsnap.com