Flame detection method based on improved ViBe algorithm and lightweight convolutional network
A technology of flame detection and convolutional network, which is applied in the field of flame detection of ViBe algorithm and lightweight convolutional network, can solve the problems of difficult flame area detection tasks, slow detection speed, difficult training, etc., to ensure speed and improve speed , The effect of improving the accuracy of flame detection
- Summary
- Abstract
- Description
- Claims
- Application Information
AI Technical Summary
Problems solved by technology
Method used
Image
Examples
Embodiment Construction
[0024] Below in conjunction with accompanying drawing and specific embodiment the case is further described:
[0025] Such as figure 1 As shown, this method of flame area detection based on surveillance video mainly includes two steps, namely the improved ViBe foreground detection algorithm and the lightweight flame detection convolutional neural network based on the Squeeze-and-Excitation (SE) attention mechanism. Network construction. Specifically, it includes the following steps:
[0026] Step 1, utilize the improved ViBe prospect detection algorithm to carry out the prospect detection of flame, concrete steps are:
[0027] Step 1.1 selects the first frame of the video sequence, and utilizes the traditional ViBe algorithm to initialize the background model;
[0028] Step 1.2 defines the flickering degree of pixels in the video frame, as shown in equation (1):
[0029] BL t (x,y)=α×BL t-1 (x,y)+β×S or (x,y) (1)
[0030] Among them, BL t (x, y) indicates the degree o...
PUM
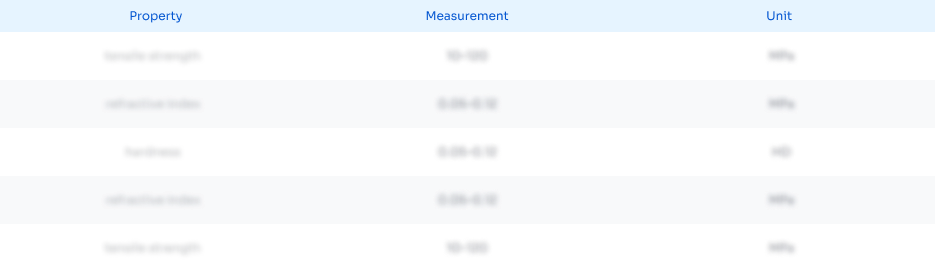
Abstract
Description
Claims
Application Information
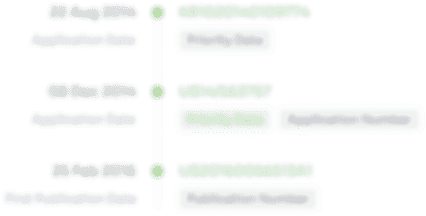
- Generate Ideas
- Intellectual Property
- Life Sciences
- Materials
- Tech Scout
- Unparalleled Data Quality
- Higher Quality Content
- 60% Fewer Hallucinations
Browse by: Latest US Patents, China's latest patents, Technical Efficacy Thesaurus, Application Domain, Technology Topic, Popular Technical Reports.
© 2025 PatSnap. All rights reserved.Legal|Privacy policy|Modern Slavery Act Transparency Statement|Sitemap|About US| Contact US: help@patsnap.com