A Prediction Method of Drilling Loss Probability Based on Naive Bayesian Algorithm
A Bayesian algorithm and prediction method technology, applied in prediction, computer parts, computing and other directions, can solve the problems of difficult to control leakage, unable to control missing parameters, repeated occurrence and so on.
- Summary
- Abstract
- Description
- Claims
- Application Information
AI Technical Summary
Problems solved by technology
Method used
Image
Examples
example 10
[0056] 1-4) In the embodiment of this description, the drilling loss probability prediction model based on the drilling loss parameters using the Naive Bayesian algorithm is a supervised learning process. In order to avoid the additional error caused by data division and affect the accuracy of the final classification results, attention should be paid to maintaining the consistency of data distribution when dividing data. At the same time, in order to ensure the representativeness of data, the division data is divided into Training set and test set, the above-mentioned preprocessed drilling loss parameter data are randomly stratified and divided into training set and test set according to the preset ratio of 10:1;
[0057] 2) figure 2 It is a flow chart of drilling loss probability prediction based on naive Bayesian algorithm, such as figure 2 As shown, in the specific implementation case of the scheme of the present invention, the drilling data parameters after data collec...
PUM
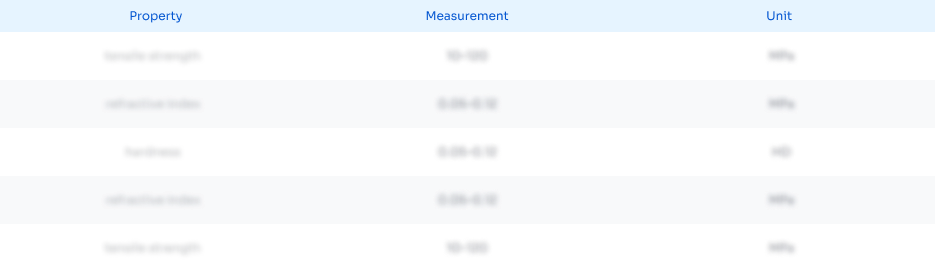
Abstract
Description
Claims
Application Information
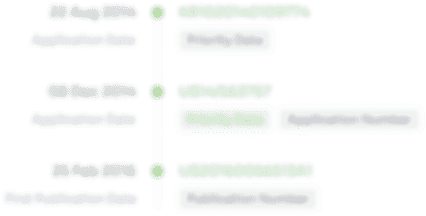
- R&D Engineer
- R&D Manager
- IP Professional
- Industry Leading Data Capabilities
- Powerful AI technology
- Patent DNA Extraction
Browse by: Latest US Patents, China's latest patents, Technical Efficacy Thesaurus, Application Domain, Technology Topic, Popular Technical Reports.
© 2024 PatSnap. All rights reserved.Legal|Privacy policy|Modern Slavery Act Transparency Statement|Sitemap|About US| Contact US: help@patsnap.com