Brain glioma segmentation model and segmentation method based on deep learning
A technique for brain glioma and segmentation models, which is applied in neural learning methods, biological neural network models, image analysis, etc., can solve problems such as high difficulty and time-wasting, and achieve the effect of improving the ability and improving the segmentation effect
- Summary
- Abstract
- Description
- Claims
- Application Information
AI Technical Summary
Problems solved by technology
Method used
Image
Examples
Embodiment 1
[0036] A brain glioma segmentation model based on deep learning of the present invention includes an encoding model and a decoding model. The encoding model includes a convolutional layer and N encoding modules. The encoding includes a hole dense unit and a pool located at the output of the hole dense unit. The above-mentioned N encoding modules are connected in sequence and located at the output end of the convolutional layer, the encoding module located at the end of the transmission is the end encoding module, and the other encoding modules are intermediate encoding modules, and N is greater than or equal to 3; the decoding module includes sequentially connected first An end decoding module, an intermediate decoding module, and an end decoding module; the head end decoding module includes sequentially connected hole dense units and upsampling convolutional layers, the head end decoding module is located at the output end of the end encoding module, and the output end of the h...
Embodiment 2
[0042] The glioma segmentation method based on deep learning of the present invention comprises the following steps:
[0043] S100. Acquiring various three-dimensional nuclear magnetic resonance images as input images, where the above nuclear magnetic resonance images include various MRI modalities and brain glial regions marked by experts;
[0044] S200. Perform normalization processing on the above-mentioned input image, so that the input image conforms to a normal distribution;
[0045] S300. Construct the glioma segmentation model based on deep learning disclosed in Example 1;
[0046]S400. Based on the normalized input image, train the above-mentioned glioma segmentation model to obtain a trained glioma segmentation model;
[0047] S500. Acquiring a nuclear magnetic resonance image to be detected as a test image, the nuclear magnetic resonance image including various MRI modalities and brain glial regions marked by experts;
[0048] S600. Perform normalization processin...
PUM
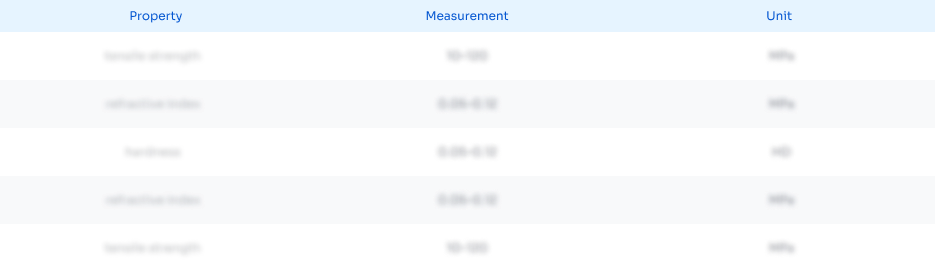
Abstract
Description
Claims
Application Information
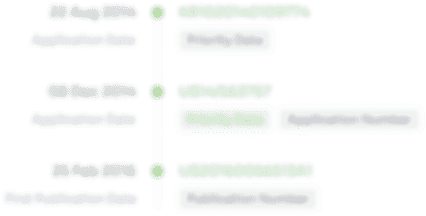
- R&D Engineer
- R&D Manager
- IP Professional
- Industry Leading Data Capabilities
- Powerful AI technology
- Patent DNA Extraction
Browse by: Latest US Patents, China's latest patents, Technical Efficacy Thesaurus, Application Domain, Technology Topic, Popular Technical Reports.
© 2024 PatSnap. All rights reserved.Legal|Privacy policy|Modern Slavery Act Transparency Statement|Sitemap|About US| Contact US: help@patsnap.com