Emergency vehicle hybrid lane changing decision-making method based on reinforcement learning and avoidance strategies
An emergency vehicle and reinforcement learning technology, applied in neural learning methods, biological neural network models, control devices, etc., can solve the problems of not considering the influence of normal traffic flow, difficult generalization, and not making full use of real-time traffic data
- Summary
- Abstract
- Description
- Claims
- Application Information
AI Technical Summary
Problems solved by technology
Method used
Image
Examples
Embodiment 1
[0172] The following is a detailed description of the effect of the present invention on the decision-making of intelligent network emergency vehicle sections through specific examples:
[0173] 1. First of all, the reinforcement learning part of the algorithm has achieved a good convergence effect, such as Figure 4 As shown, it is described that the effect of the loss function value tending to zero after nearly 200,000 steps of training is significant;
[0174] 2. During the training process, monitor the speed convergence of the DQN strategy and the "DQN+avoidance" hybrid strategy, such as Figure 5 As shown, all can converge to a lower transit time than the baseline (the default car-following model: shown by the dotted line in the figure);
[0175] 3. The mixed strategy should have been more stable, but if Figure 5 It can be seen that this is not the case, and often occurs as Figure 6 In the scenario shown, the vehicle in front continues to perform evasive actions due ...
PUM
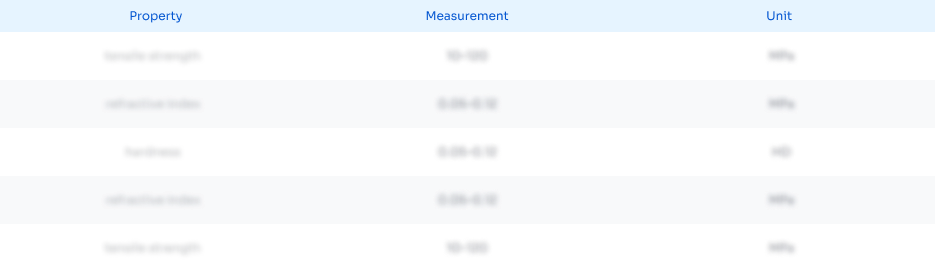
Abstract
Description
Claims
Application Information
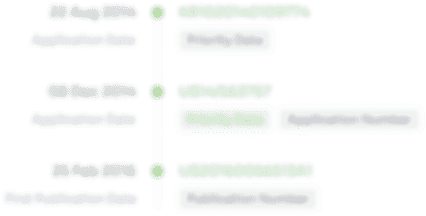
- R&D
- Intellectual Property
- Life Sciences
- Materials
- Tech Scout
- Unparalleled Data Quality
- Higher Quality Content
- 60% Fewer Hallucinations
Browse by: Latest US Patents, China's latest patents, Technical Efficacy Thesaurus, Application Domain, Technology Topic, Popular Technical Reports.
© 2025 PatSnap. All rights reserved.Legal|Privacy policy|Modern Slavery Act Transparency Statement|Sitemap|About US| Contact US: help@patsnap.com