Mechanical fault intelligent diagnosis method for implicit excitation adversarial training under small sample
A technology for intelligent diagnosis and mechanical faults, applied in mechanical bearing testing, neural learning methods, computer parts, etc., which can solve the problems of insufficient model training, limited model training data, and low detection accuracy of fault diagnosis models.
- Summary
- Abstract
- Description
- Claims
- Application Information
AI Technical Summary
Problems solved by technology
Method used
Image
Examples
Embodiment Construction
[0056] In order to make the purpose, technical solution and technical characteristics of the present invention clearer, the present invention will be further described in detail below in combination with specific implementation cases and with reference to the accompanying drawings. It should be noted that the specific implementation cases described here are only used to explain related inventions, rather than to limit the present invention.
[0057] An intelligent diagnosis method for mechanical faults with implicit incentive confrontation training under small samples, see figure 1 , including the following steps:
[0058] Step S1: Obtain one-dimensional signal data under various working conditions of mechanical equipment, divide training sample set and test sample set, and assign category label information to each training sample and test sample;
[0059] Step S2: Pseudo sample generation and feature encoding, input training samples into the encoder to obtain low-dimensional...
PUM
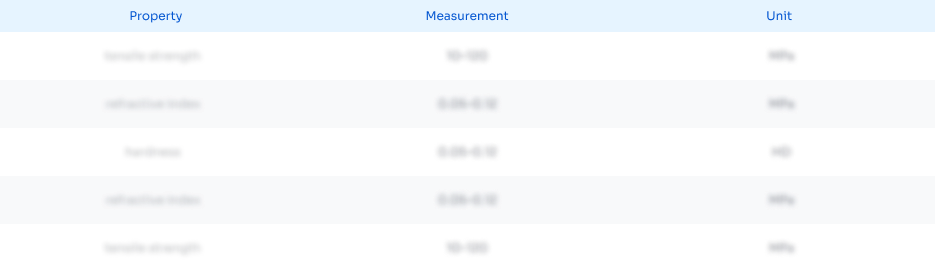
Abstract
Description
Claims
Application Information
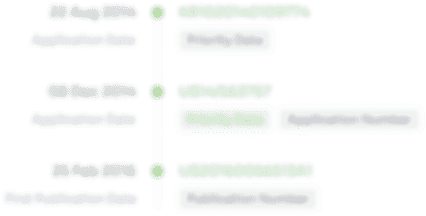
- R&D Engineer
- R&D Manager
- IP Professional
- Industry Leading Data Capabilities
- Powerful AI technology
- Patent DNA Extraction
Browse by: Latest US Patents, China's latest patents, Technical Efficacy Thesaurus, Application Domain, Technology Topic, Popular Technical Reports.
© 2024 PatSnap. All rights reserved.Legal|Privacy policy|Modern Slavery Act Transparency Statement|Sitemap|About US| Contact US: help@patsnap.com