Quantum deep reinforcement learning control method of doubly-fed wind generator
A technology for wind power generators and reinforcement learning, which is applied in neural learning methods, wind power generation, control generators, etc., and can solve problems such as increasing the time complexity of algorithms
- Summary
- Abstract
- Description
- Claims
- Application Information
AI Technical Summary
Problems solved by technology
Method used
Image
Examples
Embodiment Construction
[0084] A quantum deep reinforcement learning control method for a doubly-fed wind power generator proposed by the present invention is described in detail in conjunction with the accompanying drawings as follows:
[0085] figure 1 It is a deep learning convolutional neural network structure diagram of the present invention. The structure is mainly composed of input layer, convolution layer, pooling layer and output layer. The deep learning of the present invention adopts backpropagation algorithm to carry out training, and this method can compare the result (prediction result) calculated by the forward propagation neural network with the real label (z) to obtain an error, and then use the error of backpropagation , calculate the derivative of each neuron (weight), and start backpropagation to modify their respective weights. The specific steps are: the input layer inputs the initial input value, weight coefficient, bias and other parameters, multiplies the input value and we...
PUM
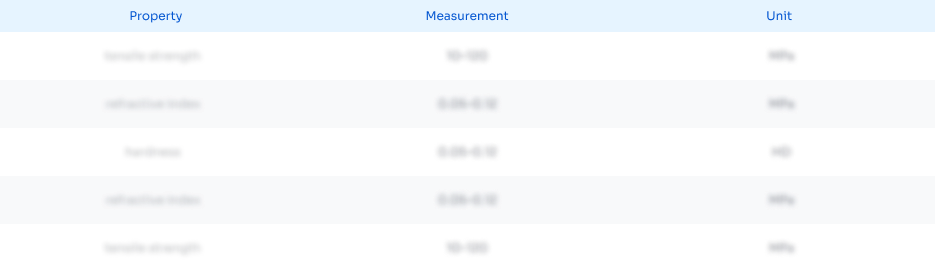
Abstract
Description
Claims
Application Information
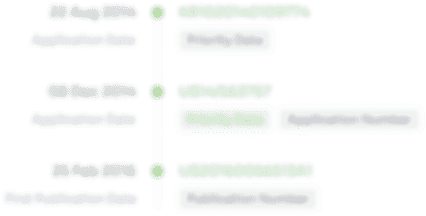
- R&D Engineer
- R&D Manager
- IP Professional
- Industry Leading Data Capabilities
- Powerful AI technology
- Patent DNA Extraction
Browse by: Latest US Patents, China's latest patents, Technical Efficacy Thesaurus, Application Domain, Technology Topic.
© 2024 PatSnap. All rights reserved.Legal|Privacy policy|Modern Slavery Act Transparency Statement|Sitemap