Double-flow convolution behavior recognition method based on 3D time flow and parallel spatial flow
A recognition method and spatial flow technology, applied in character and pattern recognition, neural learning methods, instruments, etc., can solve the problems of high storage and calculation costs of optical flow images, insufficient accuracy for practical scenarios, and feature information extraction that needs to be improved, etc. problems, to achieve the effect of improving prediction accuracy, improving recognition accuracy, and reducing recognition error probability
- Summary
- Abstract
- Description
- Claims
- Application Information
AI Technical Summary
Problems solved by technology
Method used
Image
Examples
Embodiment Construction
[0046] The following will clearly and completely describe the technical solutions in the embodiments of the present invention with reference to the drawings in the embodiments of the present invention.
[0047] The present invention provides a dual-stream convolution behavior recognition method based on 3D time stream and parallel spatial stream, such as figure 1 As shown, the specific examples are as follows:
[0048] 1. Video processing
[0049] (1) For the input video, a plurality of positive sequence video frames are randomly selected for optical flow extraction to form multiple optical flow blocks, as follows:
[0050] Randomly select 8 frames of video frames from the input video, and perform two-way optical flow extraction on these 8 frames of pictures, and stack them in order to obtain 8 optical flow blocks with 8 frames of optical flow graphs. The calculation method of optical flow extraction is as follows:
[0051]
[0052] in,
[0053] u=[1:w],v=[1:h],k=[-L+1:L...
PUM
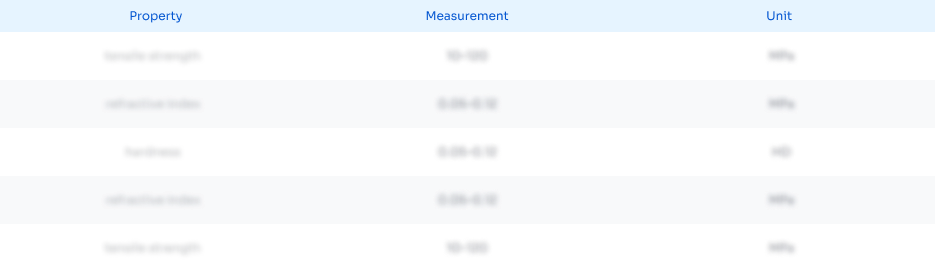
Abstract
Description
Claims
Application Information
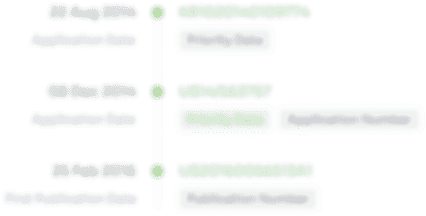
- R&D Engineer
- R&D Manager
- IP Professional
- Industry Leading Data Capabilities
- Powerful AI technology
- Patent DNA Extraction
Browse by: Latest US Patents, China's latest patents, Technical Efficacy Thesaurus, Application Domain, Technology Topic, Popular Technical Reports.
© 2024 PatSnap. All rights reserved.Legal|Privacy policy|Modern Slavery Act Transparency Statement|Sitemap|About US| Contact US: help@patsnap.com