Novel convolutional neural network model and application
A convolutional neural network and model technology, applied in the new convolutional neural network model and application field, can solve the problems of low diagnostic accuracy and difficulty, and achieve good classification ability
- Summary
- Abstract
- Description
- Claims
- Application Information
AI Technical Summary
Problems solved by technology
Method used
Image
Examples
Embodiment 1
[0050] Such as Figure 1-3 As shown, a new convolutional neural network model is based on the convolutional neural network, the improved threshold function is used as the activation function tReLU, and residual neurons are introduced into the middle layer of the convolutional neural network. Alternate connections, softmax classification, throughout the entire structure to generate a new convolutional neural network model RLCNN; the activation function tReLU is shown in formula (1):
[0051]
[0052] The residual neuron is represented by formula (2):
[0053] F(x)=W 2 f(W 1 x+b)+b (2)
[0054] In formula (2), x represents the input of the current layer, F(x) represents the input of the next layer, W 1 , W 2 Represents the weights of the current layer and the next layer, f(.) represents the tReLU activation function, and b represents the bias.
[0055] The new convolutional neural network model is a six-layer convolutional neural network, in which the convolution kernel...
Embodiment 2
[0064] Utilize the novel convolutional neural network model of the present invention to diagnose the motor bearing fault, verify the accuracy of the RLCNN model of the present invention to the bearing fault diagnosis, the basic process of the RLCNN model fault diagnosis is as follows Figure 4 shown.
[0065] 1. Dataset
[0066] The RLCNN model of the present invention is verified by using the bearing data set of Case Western Reserve University. 3 types, including outer ring fault (OF), rolling element fault (RF), and inner ring fault (IF), each type has 3 damage degrees, and the damage diameter is 0.18mm, 0.36mm, 0.54mm, a total of 9 One fault type, plus one normal (NO) type, respectively used to represent IF0.18, IF0.36, IF0.54, OF0.18, OF0.36, OF0.54, RF0.18, RF0.36, RF0 9 types of failures of .54 bearings, NO means normal. The data were recorded under 4 load conditions (0hp, 1hp, 2hp, 3hp), with 2000 vibration images for each load in the training dataset and 400 vibrati...
Embodiment 3
[0075] Utilize the novel convolutional neural network model of the present invention to diagnose the bearing fault of the electromechanical transmission system, verify the accuracy of the RLCNN model of the present invention to the bearing fault diagnosis, and the basic flow of the RLCNN model fault diagnosis is as follows Figure 4 shown.
[0076] 1. Dataset
[0077] The application of the RLCNN model of the present invention in bearing fault diagnosis is analyzed by using the bearing data set provided by Paderborn University in Germany. Select a part of the data set for training and testing. In the data set, there are artificial damage and real damage (produced by the accelerated life experiment). In the real damage, select 5 kinds of damage in the inner ring, pitting multiple damage level 1 (KI04), There are 6 fault types including single pitting damage level 3 (KI16), repeated pitting damage level 1 (KI17), single pitting damage level 2 (KI18), single pitting damage level...
PUM
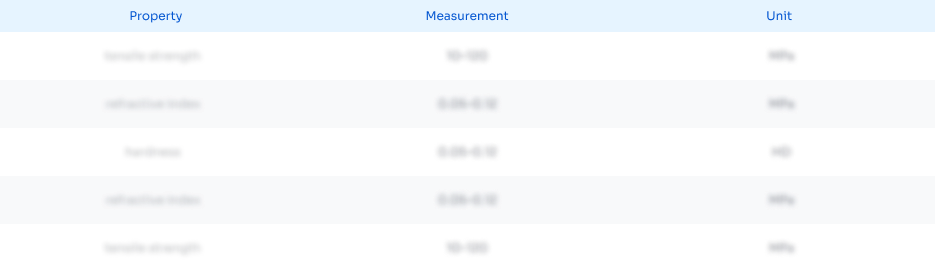
Abstract
Description
Claims
Application Information
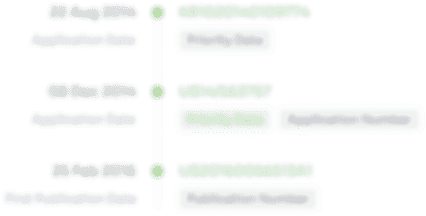
- Generate Ideas
- Intellectual Property
- Life Sciences
- Materials
- Tech Scout
- Unparalleled Data Quality
- Higher Quality Content
- 60% Fewer Hallucinations
Browse by: Latest US Patents, China's latest patents, Technical Efficacy Thesaurus, Application Domain, Technology Topic, Popular Technical Reports.
© 2025 PatSnap. All rights reserved.Legal|Privacy policy|Modern Slavery Act Transparency Statement|Sitemap|About US| Contact US: help@patsnap.com