Crop nitrogen nutrition index estimation model evaluation method based on digital image
A nutrient index and estimation model technology, which is applied in the evaluation field of crop nitrogen nutrient index estimation model, can solve problems such as difficulty in acquiring field test data, influence of model evaluation accuracy, interference, etc., and achieve accurate evaluation of generalization performance and model performance. Evaluate robust, mitigating effects
- Summary
- Abstract
- Description
- Claims
- Application Information
AI Technical Summary
Problems solved by technology
Method used
Image
Examples
Embodiment 1
[0047] A simple nonlinear regression modeling method was adopted, and the aboveground dry matter (DM), aboveground nitrogen accumulation (NA), and leaf area index (LAI) were used as the dependent variables of the model, and the image feature parameters (CC, R, G, B.L * 、a * , b * , GMR, VI Green , NRI, NGI, NBI and INT) as independent variables, using allometric growth function and exponential function for fitting, the fitting equations are: y=a+bx c and y = ae b x. Most of the image feature parameters have significant correlations with rice nitrogen nutrition indicators shoot dry matter (DM), nitrogen accumulation (NA) and leaf area index (LAI), among which CC, b * , NGI, VI Green , GMR were positively correlated with DM, LAI, NA, and the other image characteristic parameters were negatively correlated with the three nitrogen nutrition indexes. In addition, there are large differences in the correlation coefficients between different types of image feature parameters. ...
Embodiment 2
[0057] Using BP neural network (BPNN) to estimate rice nitrogen nutrition parameters, a total of 21 BPNNs were trained, and the output variables were dry matter (DM), nitrogen accumulation (NA) and leaf area index (LAI). A nitrogen nutrition index, the input variable is 7 different image parameter combinations, and it is evaluated by the algorithm. Combinations of these 7 input variables are named M1-M7: M1, CC; M2, VI Green ; M3, GMR; M4, including CC, VI Green 3 image indexes including , GMR; M5, 7 image indexes (CC, L * 、a * , b * , R, G, B); M6, 8 image metrics extracted from non-segmented images (GMR, VI Green , L * 、a * , b * , R, G, B); 15 image indicators in M7, M5 and M6.
[0058] The data set is divided into three parts according to the ratio of 70:15:15 for BPNN training, verification and testing. The training dataset is used to fit the weights and biases of the BPNN. The validation dataset is used to prevent overfitting: the error of the validation datase...
Embodiment 3
[0065] Using random forest (RF) regression to estimate rice nitrogen nutrition parameters, seven image indices (CC, L * 、a * , b * , R, G, B) and 8 image indices (GMR, VI Green , L * 、a * , b * , R, G, B) as input variables for RF regression. In order to find the optimal number of decision trees (ntree) for the estimation of aboveground dry matter (DM), aboveground nitrogen accumulation (NA) and leaf area index (LAI), tests were carried out between 30 and 1000 with 10 as the interval. The number of variables considered (mtry) for each branch node in the decision tree is set to 5 (1 / 3 of the total input variables). Other hyperparameters in RF regression are set to default values according to the "Random Forest Regressor" function in the scikit-learn library. The importance of an input variable is calculated by Gini impurity, which is expressed as the proportion of this variable to the total contribution of all variables in the model, and the sum of the importance of al...
PUM
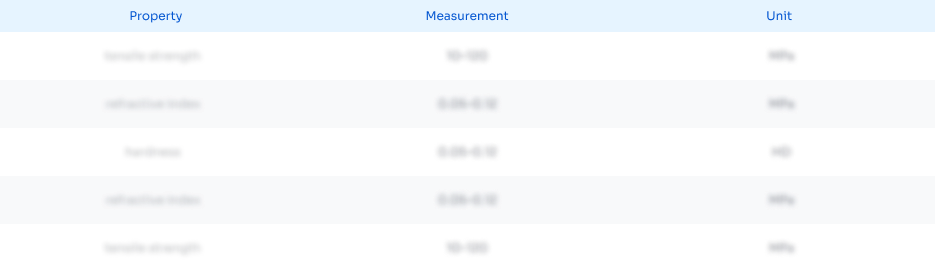
Abstract
Description
Claims
Application Information
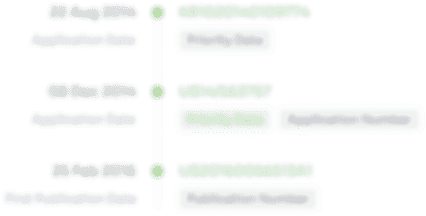
- R&D Engineer
- R&D Manager
- IP Professional
- Industry Leading Data Capabilities
- Powerful AI technology
- Patent DNA Extraction
Browse by: Latest US Patents, China's latest patents, Technical Efficacy Thesaurus, Application Domain, Technology Topic, Popular Technical Reports.
© 2024 PatSnap. All rights reserved.Legal|Privacy policy|Modern Slavery Act Transparency Statement|Sitemap|About US| Contact US: help@patsnap.com